Presented at Activate 2018
Slides: [ Ссылка ]
There has been much progress in AI thanks to advances in deep learning in recent years, especially in areas such as computer vision, speech recognition, natural language processing, playing games, robotics, machine translation, etc. This presentation aims at introducing some of the core concepts and motivations behind deep learning and representation learning. Deep learning builds on many of the ideas introduced decades earlier with the connectionist approach to machine learning, inspired by the brain. These essential early contributions include the notion of distributed representation and the back-propagation algorithm for training multi-layer neural networks, but also the architecture of recurrent neural networks and convolutional neural networks. In addition to the substantial increase in computing power and dataset sizes, many modern additions have contributed to the recent successes. Thanks to soft-attention mechanisms neural nets have moved from pattern recognition devices working on vectors to general-purpose differentiable modular machines which can handle arbitrary data structures. The talk will end with a discussion of some major open problems for AI which are at the forefront of research in deep learning and reinforcement learning.
Learn more: [ Ссылка ]
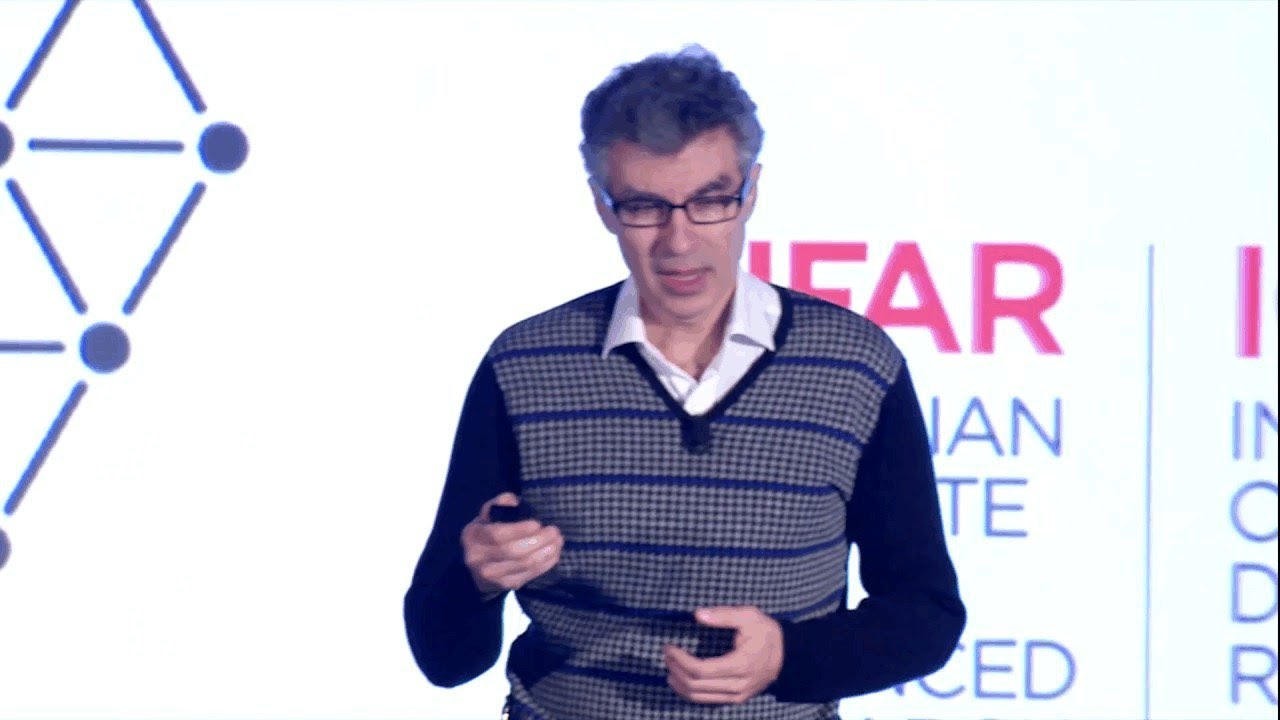