Imagine there is a facial recognition system that’s been put in place by the government (or Kmart, or Bunnings), that is purely being used to detect known criminals. As soon as a criminal is detected, police are automatically called out and arrest the individual to take them back to the station for further questioning. Sounds good, doesn’t it? Well, I suppose some of you are a bit sceptical…
Books on Paradoxes: [ Ссылка ] (affiliate link)
“It is a paradox that every dictator has climbed to power on the ladder of free speech. Immediately on attaining power each dictator has suppressed all free speech except his own.”
~ Herbert Hoover (1874–1964), 31st U.S. President
To help put your mind at ease, let’s say that this technology is highly accurate. Let’s say it’s 99% accurate. That means if the system scans a criminal, it will correctly identify the criminal 99% of the time, but it will fail 1% of the time (the false negative rate). On the flip side, if an innocent person is walking past, the system will correctly identify them as a non-criminal 99% of the time, but it will incorrectly identify them as a criminal 1% of the time (the false positive rate), hence why the police need to question every person who comes in, because there’s a 1% chance they’ve arrested an innocent person. Let’s assume that criminals are pretty stupid and don’t try to disguise themselves, or wear some sort of face covering to fool the system. It would be a bit strange, anyway, right, people walking around in public with their faces covered?
So for this system that is 99% accurate, imagine a member of the public is walking past one of these cameras and is detected as a criminal. What do you think is the chance, or the probability, that this person is actually a criminal? 99%, right? (Or approximately 99%). I mean, the facial recognition software is 99% accurate. Well, although this inference seems to make sense, it is actually flawed reasoning. What if I told you that it was actually much, much less than that. What if I told you it was actually closer to 1%? Let me explain through use of an example.
Imagine this system has been implemented in a large city, and every day, 1,000,000 faces are scanned successfully. Let’s say that 100 of those people are actually criminals (so about one in ten thousand people). The highly accurate facial recognition system successfully detects 99 of those 100 criminals and the police are called out and make 99 successful arrests. 99 criminals are now off the streets thanks to this technology. Yeah, science! But wait! Of those million faces that were scanned, 999,900 of them were innocent people. However, we have to remember that this system isn’t perfect and has a 1% false positive rate. So this means that of those 999,900 innocent people who had their faces scanned just because they happened to be walking past a camera, 9,999 of them will be falsely detected as being a criminal and will consequently be arrested. Therefore, throughout the day, a total of 10,098 people will be detected as criminals, among which only 99 are actual criminals. So, the probability that a person detected by the system is a criminal is only about 99 in 10,098, which is less that 1%, and very, very far below our initial guess of 99%.
This is called the Base Rate Fallacy, or the False Positive Paradox, and is so misleading in this example because there are many more innocent people than there are criminals, and the number of false positives (that is, innocent people scanned as criminals) is so much larger than the true positives (actual criminals scanned as criminals).
The point of this, is that we have to be very careful with the stated accuracy of certain technology and tests, things such as tests for disease and so on. Just say a particular test for a particular disease has an accuracy of say 90% (still fairly high). Just say that in a population of 1,000,000 people, 2% of people actually have the disease (20,000 people). So the test correctly identifies 18,000 people who actually have the disease (presuming that all these people go and get tested). However, if all the rest of the population (980,000 people) also get tested, because the government told them to, or whatever, then 10% of them will also test positive even though they’re not actually positive. That means 98,000 people will falsely test positive. This gives a total of 116,000 people who tested positive.
So in this fictional example, only 18,000 of the 116,000 total people with a positive test result are actually infected. So, the probability of actually being infected after one is told that one is infected is only about 15.5% for a test that otherwise appears to be “90% accurate”!
MUSIC
Melancholia by Godmode
#falsepositive #paradox #facialrecognition #fallacies
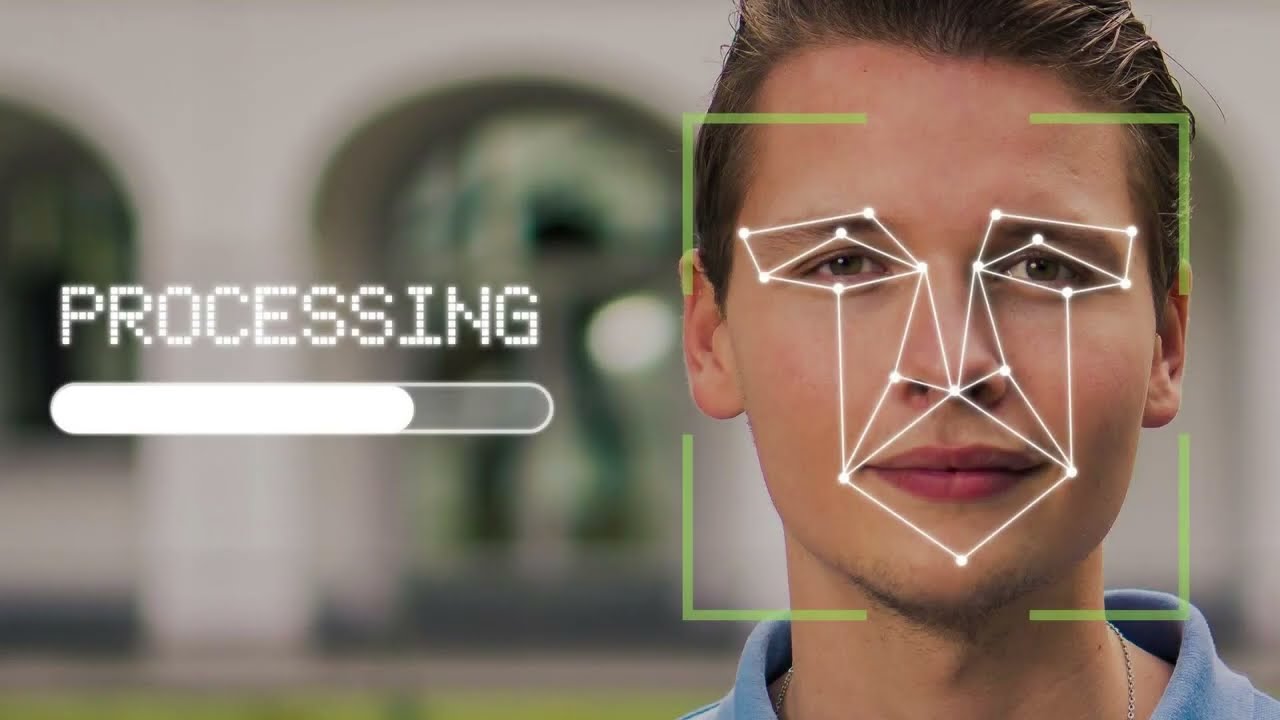