"AI at the Bench: Legal and Ethical Challenges of Informing – or Misinforming – Judicial Decision-Making Through Generative AI"
---------------------------------------------------------------------------------
OVERVIEW:
📋 This paper provides a systematic review of existing AI regulations in Europe, the United States, and in Canada. Based on the analysis, the paper suggests that there are three main regulatory strategies for AI: AI-focused overhauls of existing regulation, the introduction of novel AI regulation, and the omnibus approach.
---------------------------------------------------------------------------------
Submission: DAP-2023-0148
AUTHORS:
Nydia Remolina
Hitotsubashi University, Hitotsubashi Institute for Advanced Study, Graduate School of Law, Tokyo, Japan,
David Socol de la Ossa
Singapore Management University, Singapore; Fintech Track Lead, SMU Centre for AI and Data Governance, Singapore.
---------------------------------------------------------------------------------
Follow Data for Policy here:
[ Ссылка ]
Subscribe: [ Ссылка ]
Linkedin: [ Ссылка ]
[ Ссылка ]
Sign up for Newsletters: [ Ссылка ]
#generativeai #genai #decisionmaking
---------------------------------------------------------------------------------
ABSTRACT:
In this paper, we provide a systematic review of existing AI regulations in Europe, the United States, and in Canada. We build on the qualitative analysis of 129 AI regulations (enacted and not enacted) to identify patterns in regulatory strategies and in AI transparency requirements. Based on the analysis of this sample, we suggest that there are three main regulatory strategies for AI: AI-focused overhauls of existing regulation, the introduction of novel AI regulation, and the omnibus approach. We argue that although these types emerge as distinct strategies, their boundaries are porous as the AI regulation landscape is rapidly evolving. We find that across our sample, AI transparency is effectively treated as a central mechanism for meaningful mitigation of potential AI harms. We therefore focus on AI transparency mandates in our analysis and identify six AI transparency patterns: human in the loop, assessments, audits, disclosures, inventories, and red teaming. We contend that this qualitative analysis of AI regulations and AI transparency patterns provides a much needed bridge between the policy discourse on AI, which is all too often bound up in very detailed legal discussions, and applied socio-technical research on AI fairness, accountability, and transparency.
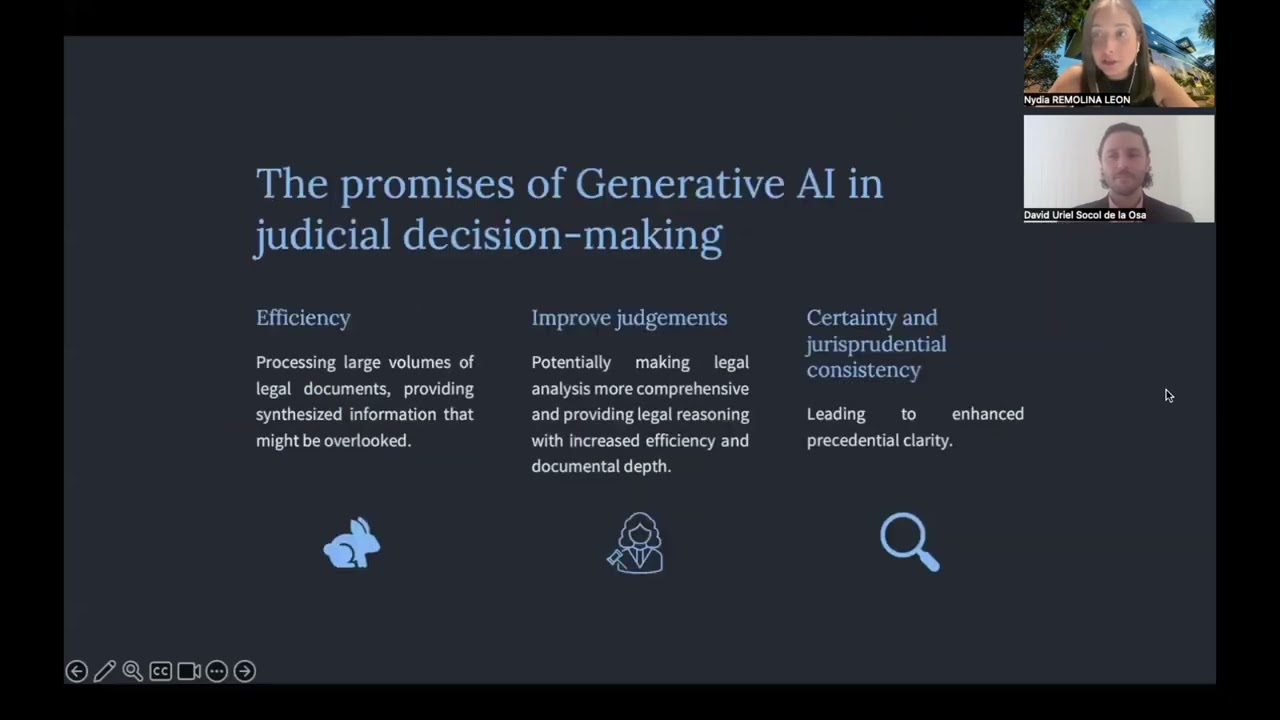