Advancements in technology, such as machine learning and AI, have provoked the question on how to best apply this technology in order to maximize the supply chain performance. Can machine learning be applied in a way that, by providing it with more and more forecasting data, increasingly better forecasts are being produced through complex feedback loops? Can a computer be made to make better supply chain decisions than humans? What is the risk of feedback loops becoming a self-fulfilling prophecy?
We are joined by Alexander Backus to discuss these questions in this episode. Mr. Backus is an expert in the field of machine learning and is the Data and Analytics leader at IKEA.
Self-fulfilling prophecies is a psychological phenomenon where a prediction or expectation of the future is coming true simply because it was assumed or anticipated to be the case. This is often the case in the supply chain industry where forecasts of the future become business goals to achieve, thus influencing all efforts to street the supply chain outcome in that direction. At Lokad we would argue that self-fulfilling prophecies are neither good nor bad, but something that is important to embrace rather than to pretend they don’t prevail.
When it comes to the application of machine learning in the supply chain, the core output of those systems should be supply chain decisions - not forecasts. Forecasts are only a prediction of the future, whereas decisions are the only thing affecting the supply chain performance. Through reinforcement learning, software can start learning the financial impact of every previously registered decision from gathered data to be able to compare all feasible decisions and output the most economically beneficial one. The main challenge is to be able to predict outcomes of decisions that have not previously been taken, especially the ones accounting for more extreme and unusual scenarios in supply chains.
******
Time stamps:
0:00:00 - Introduction
0:02:15 - What is a self-fulfilling prophecy?
0:03:00 - Do you affect the future because you thought it would be a certain way?
0:04:44 - What are the drawbacks when forecasts become future goals to reach, rather than evaluating supply chain performance?
00:05:58 - Are self-fulling prophecies neither good nor bad?
0:10:25 - What type of data is actually fed back into these machine learning algorithms?
0:14:06 - How do we avoid the zero-forecasting problem?
0:15:50 - What metric should we look away from?
0:18:53 - Can sales send a wrong signal about the demand?
0:20:09 - How can we avoid assuming zero sales to mean zero demand?
0:25:00 - How does probabilistic forecasting improve machine learning systems run on feedback loops compared to time series forecasting?
0:35:55 - Alexander, do you agree with the strength and weaknesses with probabilistic forecasts that Joannes just outlined?
0:36:51 - Is the main challenge to create a model that can accurately predict the outcome of all potential decisions?
0:42:46 - Joannes, what is your take on bypassing the chicken-egg problem by starting the machine learning process offline until it becomes good enough to be applied to an actual supply chain and starts learning online?
0:48:42 - Will the data history lack the more unusual, “outlier” scenarios for the machine learning system to consider the impact of all possible decisions?
0:50:19 - Nonetheless, even if we don’t necessarily have the whole specter of decisions and their implications recorded in the data, would learning offline still be a good starting point?
0:54:44 - Where should we street the development of this technology in the Supply Chain industry?
0:56:10 - Joannes, what is your take on how reinforcement learning through feedback loops will be adopted in the supply chain industry in the near future?
01:01:05 - Alexander, what kind of talents or specific qualities are you looking for in people that you onboard to your team at Ikea?
******
Check out our website: [ Ссылка ]
Follow us on LinkedIn: [ Ссылка ]
Follow us on Twitter: [ Ссылка ]
Read our blog: [ Ссылка ]
Sign-up for the Lokad newsletter: [ Ссылка ]
******
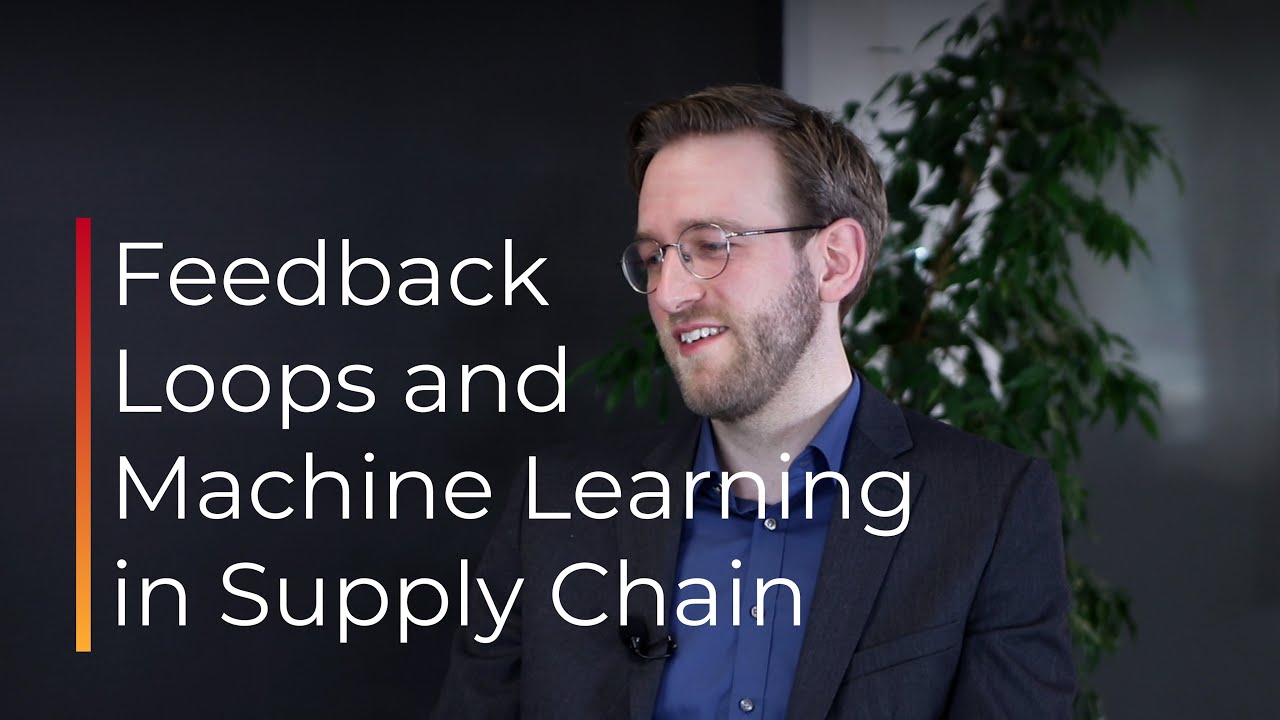