PyData Amsterdam 2017
A lot of theory is available on how the statistics of A/B testing could be improved using Bayesian statistics. In this talk I will discuss several theoretical problems and I will share my experiences on whether they actually impact A/B testing in practice. This will be demonstrated using hierarchical models build with pymc. Finally, I will share how I successfully implemented this into business.
I will first shortly discuss frequentist calculation of an A/B test, and three problems: the normal distribution instead of the beta distribution, multiple comparison problem and biased stopping times. Using these topics, I will shortly introduce Bayesian statistics and more specifically hierarchical Bayes, by using examples in pymc. I will then share whether these topics actually have direct implications for testing in practice and illustrate why several aspects hardly change the decisions made. I will then focus on one of the most important aspects from a business perspective: when to stop an insignificant test. I will present the stopping rule I currently use, explain how this works in practice and how this relates to solving the theoretical problems. 00:00 Welcome!
00:10 Help us add time stamps or captions to this video! See the description for details.
Want to help add timestamps to our YouTube videos to help with discoverability? Find out more here: [ Ссылка ]
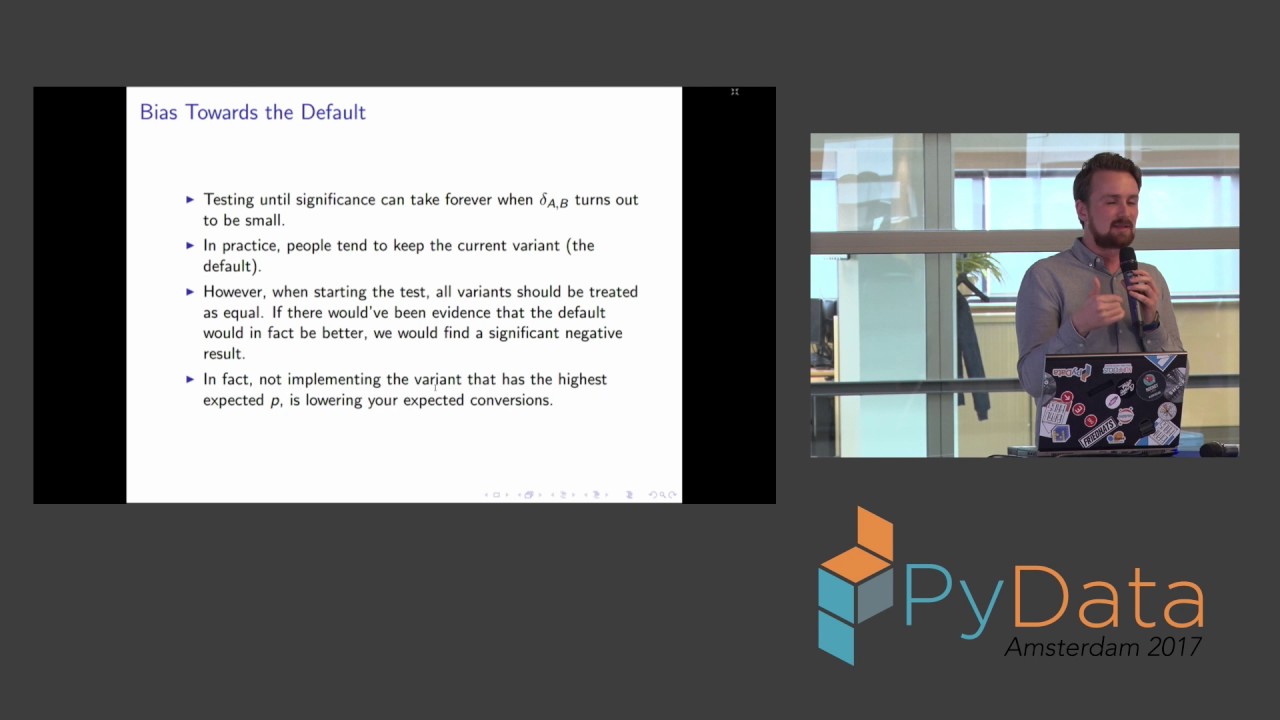