With the increase of popularity VR/AR applications, 3D hand pose estimation task has become very popular. 3D hand pose estimation from a single RGB camera has great potential, because RGB cameras are cheap and already available on most mobile devices. In this thesis we work on improving pipeline for 3D hand pose estimation from RGB camera. We dealt with two challenges - sophisticated algorithmic task and absence of good datasets. We trained several convolutional neural networks and showed that direct heatmaps method is the best approach for 2D pose estimation and vector representation - for 3D pose. We demonstrated that adding data augmentations even for synthetic dataset increases performance on real data. For 2D hand pose estimation, we proved that it is possible to train neural network on large-scale synthetic dataset and finetune it on small partly labeled real dataset to receive adequate results, even when only small part of keypoint labels is available. With no real 3D labels available, model trained on synthetic data still could correctly predict 3D keypoint locations for simple poses. All code and pre-trained models will be publicly available.
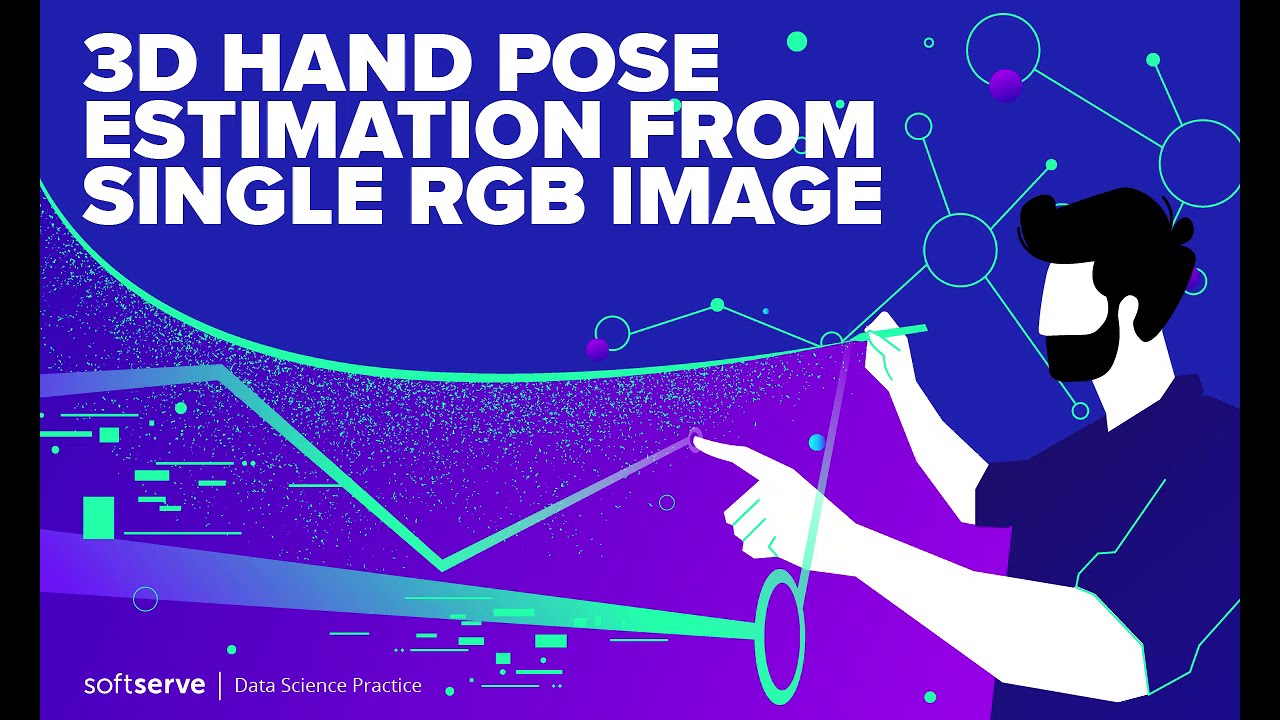