Proximal Policy Optimization (PPO) has emerged as a powerful on policy actor critic algorithm. You might think that implementing it is difficult, but in fact tensorflow 2 makes coding up a PPO agent relatively simple.
We're going to take advantage of my PyTorch code for this, as it serves as a great basis to expand on. Simply go to my github and copy the code, and then follow along.
Code for this video is here:
[ Ссылка ]
A written crash course to PPO can be found here:
[ Ссылка ]
Learn how to turn deep reinforcement learning papers into code:
Get instant access to all my courses, including the new Prioritized Experience Replay course, with my subscription service. $29 a month gives you instant access to 42 hours of instructional content plus access to future updates, added monthly.
Discounts available for Udemy students (enrolled longer than 30 days). Just send an email to sales@neuralnet.ai
[ Ссылка ]
Or, pickup my Udemy courses here:
Deep Q Learning:
[ Ссылка ]
Actor Critic Methods:
[ Ссылка ]
Curiosity Driven Deep Reinforcement Learning
[ Ссылка ]
Natural Language Processing from First Principles:
[ Ссылка ]
Reinforcement Learning Fundamentals
[ Ссылка ]
Here are some books / courses I recommend (affiliate links):
Grokking Deep Learning in Motion: [ Ссылка ]
Grokking Deep Learning: [ Ссылка ]
Grokking Deep Reinforcement Learning: [ Ссылка ]
Come hang out on Discord here:
[ Ссылка ]
Need personalized tutoring? Help on a programming project? Shoot me an email! phil@neuralnet.ai
Website: [ Ссылка ]
Github: [ Ссылка ]
Twitter: [ Ссылка ]
Time stamps:
0:00 Intro
01:17 Code restructure
01:57 PPO Memory
03:05 Network classes
08:41 Agent class
24:39 Main file
25:54 Moment of Truth
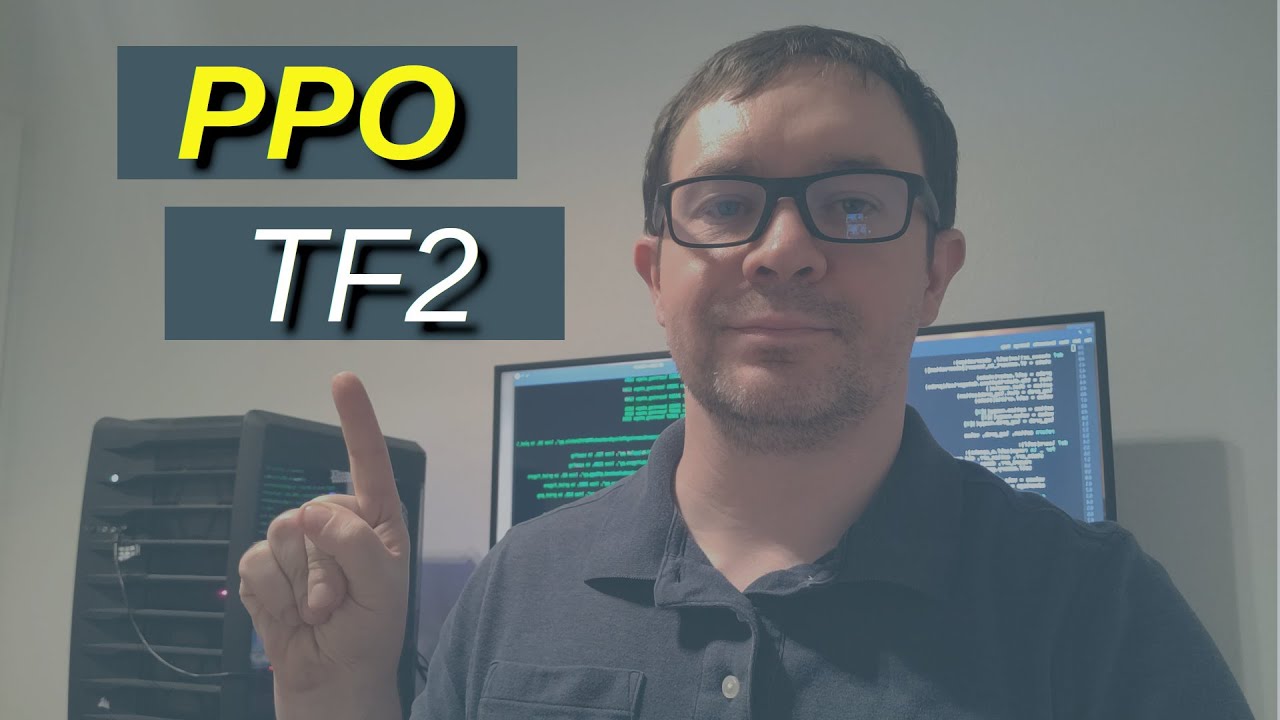