In this video we are looking at Diffusion Models from a different angle, namely through Score-Based Generative Models, which arguably can be considered as the broader family of diffusion models. Personally, this approach has helped me so much in getting a better intuition for diffusion models and how to visualize the idea and especially connect different approaches like DDPM, DDIM or EDM to one another.
00:00 Introduction
03:13 Score
04:18 Score Matching
09:10 Noise Perturbation
12:33 Denoising Score Matching
21:41 Sampling
24:00 Multiple Noise Perturbations
26:03 Differential Equations
31:36 Link to diffusion models
33:58 Summary
37:10 Conclusion
Further Reading:
1. Sliced Score Matching: [ Ссылка ]
2. Improved Techniques for Score-Based Generative Models: [ Ссылка ]
3. Generative Modeling by Estimating Gradients of the Data Distribution: [ Ссылка ]
4. Original Score Matching Paper (Hyvärinen): [ Ссылка ]
5. Langevian Dynamics: [ Ссылка ]
6. Score-Based Generative Modeling through Stochastic Differential Equations: [ Ссылка ]
7. A Connection Between Score Matching and Denoising Autoencoders: [ Ссылка ]
8. EDM: [ Ссылка ]
9. DDPM: [ Ссылка ]
10. DDIM: [ Ссылка ]
11. Yang Song Blog Post on Score Matching: [ Ссылка ]
#diffusion #scorematching #stablediffusion #maths #flux #generativemodels
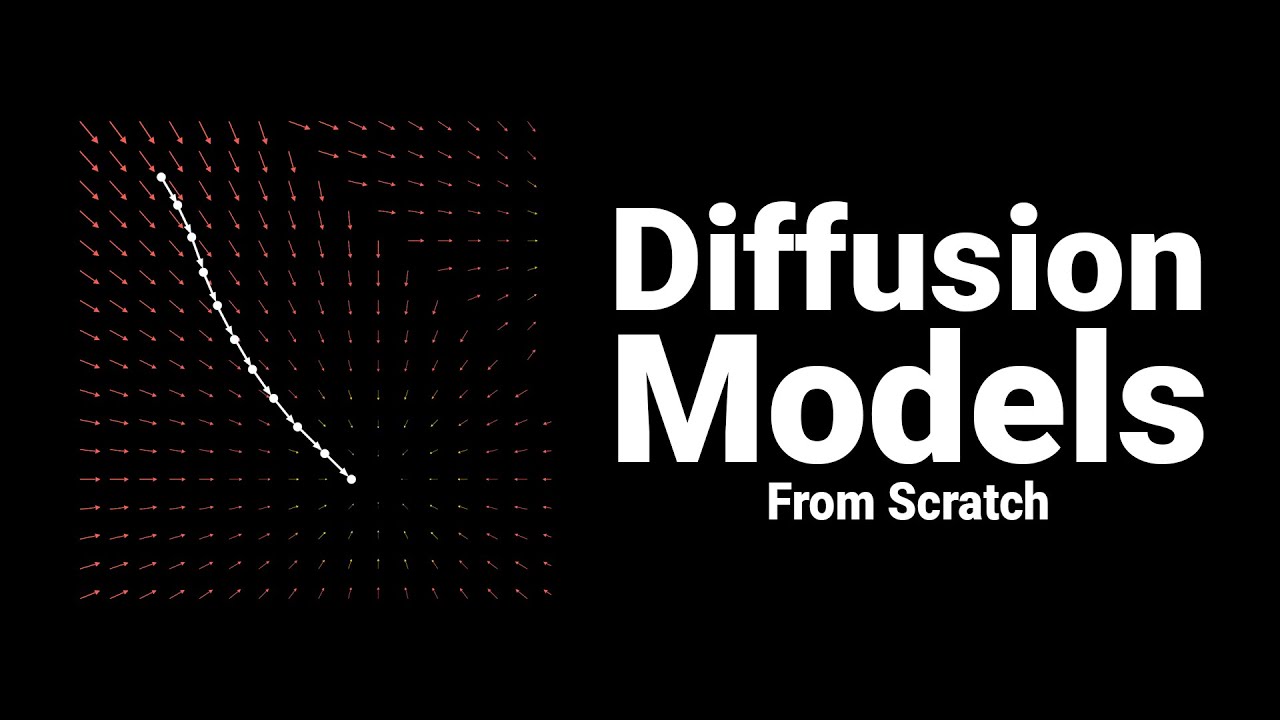