From real-time patient wait time predictions to training models on unstructured medical images, this video is covering a wide range of best practices in creating and productionizing machine learning-based solutions in healthcare.
This video session will showcase the creation of an entire ML lifecycle from data ingestion to model creation, deployment, and visualization for a healthcare scenario using Microsoft Azure services.
You will learn how to:
- Create and orchestrate data ingestion pipelines and secure storage for training and inferencing data.
- Leverage Azure Databricks and Azure Machine Learning for feature processing
- Apply AutoML in Azure Machine learning for training and hyperparameter tuning in a parallelized manner.
- Deploy and score models for both parallel batch and real-time prediction using Azure machine learning and streaming analytics.
- Assess the explainability and fairness of trained models.
We explore how to simplify the process of getting data science to production in an Azure environment while drastically cutting down the time needed to get data science to production. We’ll see how to map a healthcare business problem into an ML production pipeline through utilizing the right tools, and ultimately how to deploy ML models in production at scale to accelerate business value.
This session will also include a live demo for real-time waiting time prediction in hospitals and batch scoring to predict admitted patients' length of stay.
🔔 Subscribe for more cloud computing, data, and AI analytics videos
by clicking on subscribe button so you don't miss anything.
✅ You can contact me at:
LinkedIn:
[ Ссылка ]
Email:
mo.ghodrati95@gmail.com
#AzureML #AzureMachineLearning #MLOps #AzureMLOps #AzureDevOps #DevOpsForMachineLearning #MAchineLearningOperation #Azure #AI #DevOps
#AIinHealthcare #Healthcare #MLHealthcare #MachineLearningHealthcare #ExplainableAI #InterpretML #AiFairness #ReposibleAI #AIforHealthcare
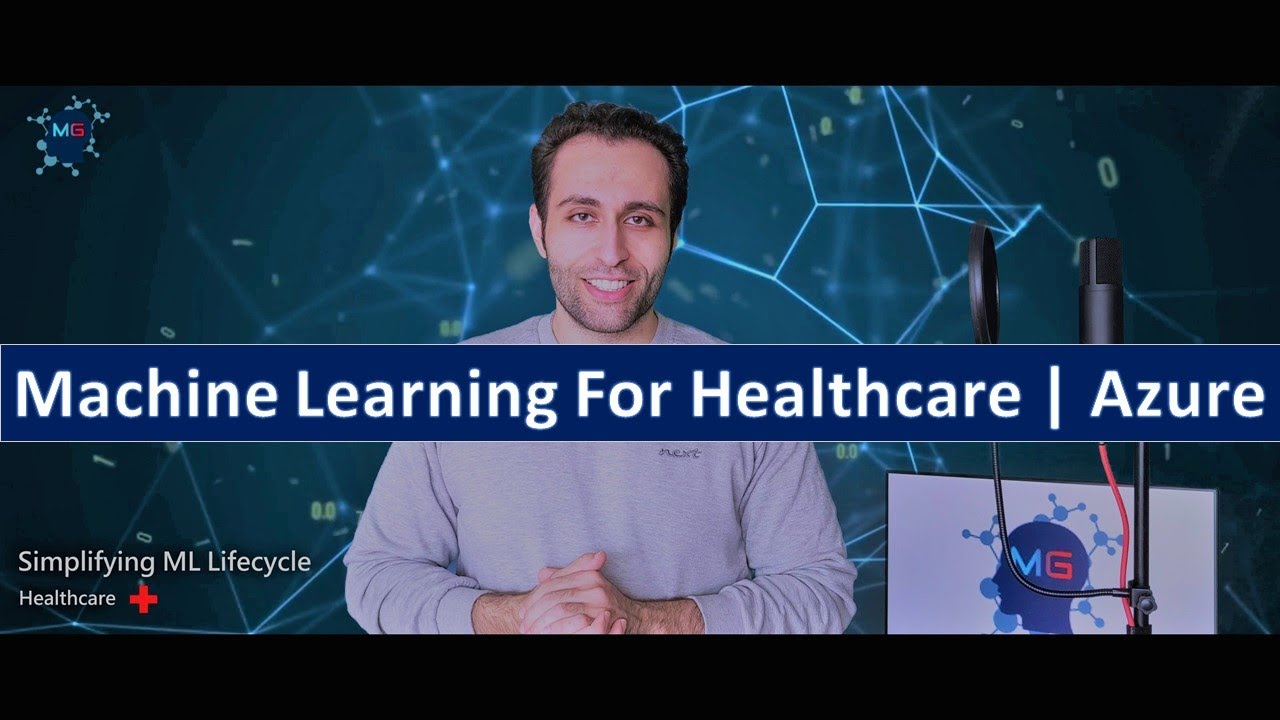