Adversarial Multi-view Networks for Activity Recognition
Lei Bai, Lina Yao, Xianzhi Wang, Salil S. Kanhere, Bin Guo, Zhiwen Yu
UbiComp '20: The ACM International Joint Conference on Pervasive and Ubiquitous Computing 2020
Session: Human Activity Recognition I
Abstract
Human activity recognition (HAR) plays an irreplaceable role in various applications and has been a prosperous research topic for years. Recent studies show significant progress in feature extraction (i.e., data representation) using deep learning techniques. However, they face significant challenges in capturing multi-modal spatial-temporal patterns from the sensory data, and they commonly overlook the variants between subjects. We propose a Discriminative Adversarial MUlti-view Network (DAMUN) to address the above issues in sensor-based HAR. We first design a multi-view feature extractor to obtain representations of sensory data streams from temporal, spatial, and spatio-temporal views using convolutional networks. Then, we fuse the multi-view representations into a robust joint representation through a trainable Hadamard fusion module, and finally employ a Siamese adversarial network architecture to decrease the variants between the representations of different subjects. We have conducted extensive experiments under an iterative left-one-subject-out setting on three real-world datasets and demonstrated both the effectiveness and robustness of our approach.
DOI:: [ Ссылка ]
WEB:: [ Ссылка ]
Remote Presentations for ACM International Joint Conference on Pervasive and Ubiquitous Computing 2020 (UbiComp '20)
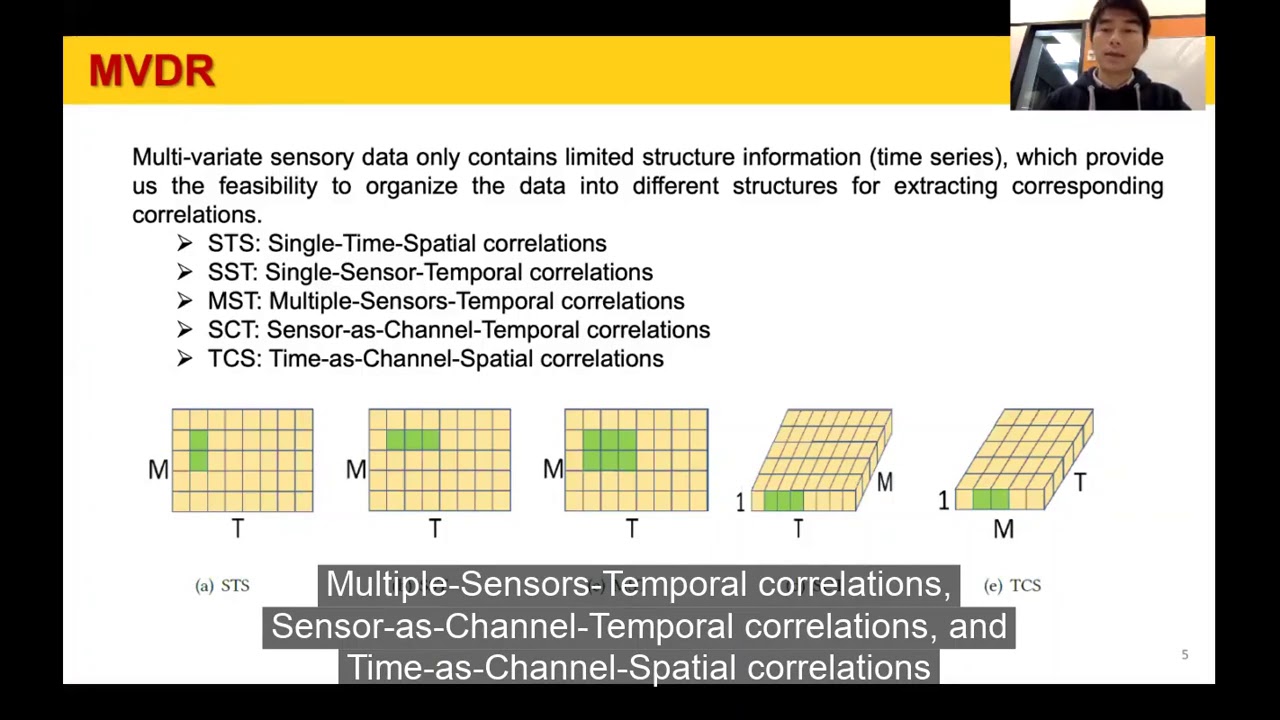