Models, Inference and Algorithms
Broad Institute of MIT and Harvard
March 24, 2021
MIA Meeting: Large-scale clinical interpretation of genetic variants using evolutionary data and deep learning
Mafalda Dias
Marks Lab, Harvard Medical School
Jonathan Frazer
Marks Lab, Harvard Medical School
Quantifying the pathogenicity of protein variants in human disease-related genes would have a profound impact on clinical decisions, yet the overwhelming majority (over 98%) of these variants still have unknown consequences. In principle, computational methods could support the large-scale interpretation of genetic variants. However, prior methods have relied on training machine learning models on available clinical labels. Since these labels are sparse, biased, and of variable quality, the resulting models have been considered insufficiently reliable. By contrast, our approach leverages deep generative models to predict the clinical significance of protein variants without relying on labels. The natural distribution of protein sequences we observe across organisms is the result of billions of evolutionary experiments. By modeling that distribution, we implicitly capture constraints on the protein sequences that maintain fitness. Our model EVE (Evolutionary model of Variant Effect) not only outperforms computational approaches that rely on labelled data, but also performs on par, if not better than, high-throughput assays which are increasingly used as strong evidence for variant classification. After thorough validation on clinical labels, we predict the pathogenicity of 11 million variants across 1,088 disease genes, and assign high-confidence reclassification for 72k Variants of Unknown Significance. Our work suggests that models of evolutionary information can provide a strong source of independent evidence for variant interpretation and that the approach will be widely useful in research and clinical settings.
Primer: Generative models of antibodies for functionally optimized library design
March 24, 2021
Jung-Eun (June) Shin
Marks Lab, Harvard Medical School
Antibodies are valuable tools for molecular biology and therapeutics because they can detect low concentrations of target antigens with high sensitivity and specificity. The increasing demand for and success with rapid and efficient discovery of novel antibodies and nanobodies using phage and yeast display methods have spurred interest in the design of optimal starting libraries. Synthetic libraries often contain a substantial fraction of non-functional proteins because current library construction methods lack higher-order sequence constraints. In order to overcome these limitations, we can design smart libraries of fit and diverse nanobodies by leveraging the information in sequences from natural repertoires and experimental assays. However, state-of-art generative models rely on sequence families and alignments, and alignment-based methods are inherently unsuitable for the statistical description of the variable length, hypermutated complementarity determining regions (CDRs) of antibody sequences, which encode the diverse specificities of binding to antigens. We developed a deep generative model adapted from natural language processing for prediction and design of diverse functional sequences without the need for alignments. By training on natural nanobody repertoires, we designed and tested a 105-nanobody library that shows better expression than a state-of-art, 1000-fold larger synthetic library. While natural repertoires contain examples of generally fit sequences, experimental assays can explicitly interrogate individual fitness features such as thermostability, poly-reactivity, and affinity, from which we can train statistical models and generate sequences optimized for each trait. With sequence models of both unlabeled natural repertoires and labeled experimental data, we can design a biased nanobody library to improve expression, stability, and capacity to bind target antigens.
For more information visit: [ Ссылка ]
Copyright Broad Institute, 2021. All rights reserved.
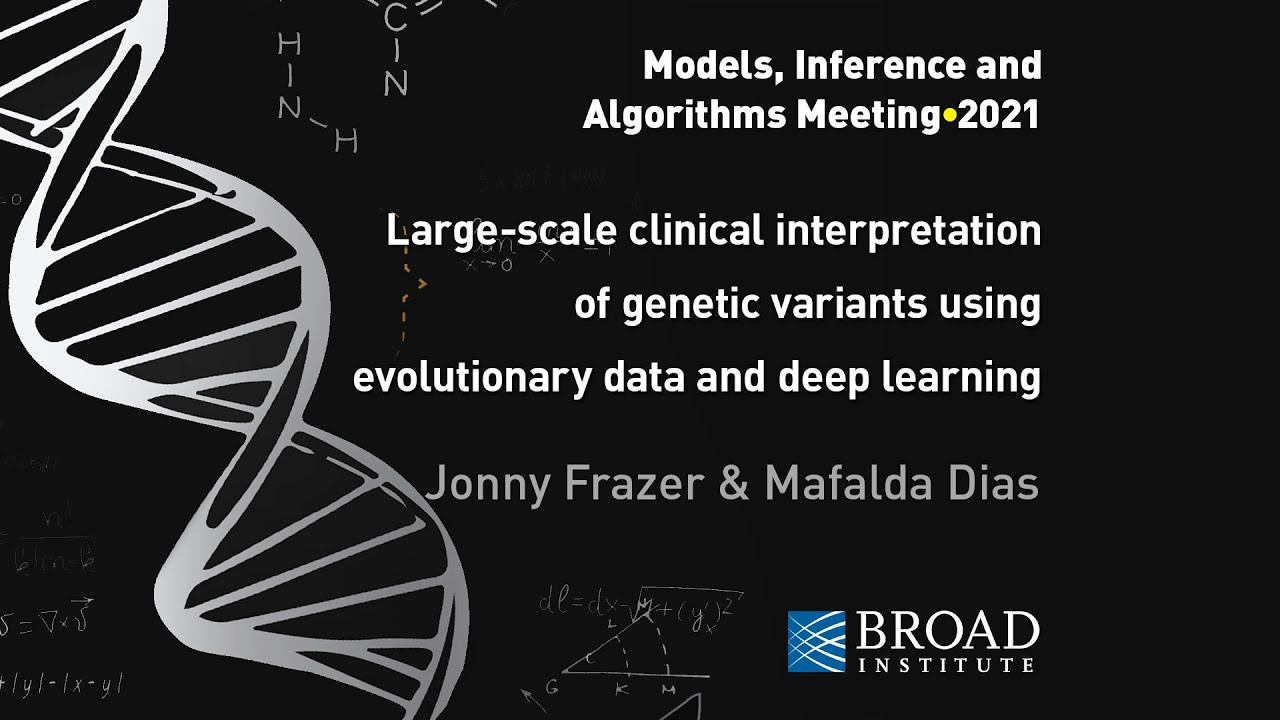