A Visual Interaction Framework for Dimensionality Reduction Based Data Exploration
Marco Cavallo, Çağatay Demiralp
CHI '18: ACM CHI Conference on Human Factors in Computing Systems
Session: Exploration through modelling and visualisation
Abstract
Dimensionality reduction is a common method for analyzing and visualizing high-dimensional data. However, reasoning dynamically about the results of a dimensionality reduction is difficult. Dimensionality-reduction algorithms use complex optimizations to reduce the number of dimensions of a dataset, but these new dimensions often lack a clear relation to the initial data dimensions, thus making them difficult to interpret. Here we propose a visual interaction framework to improve dimensionality-reduction based exploratory data analysis. We introduce two interaction techniques, forward projection and backward projection, for dynamically reasoning about dimensionally reduced data. We also contribute two visualization techniques, prolines and feasibility maps, to facilitate the effective use of the proposed interactions. We apply our framework to PCA and autoencoder-based dimensionality reductions. Through data-exploration examples, we demonstrate how our visual interactions can improve the use of dimensionality reduction in exploratory data analysis.
DOI:: [ Ссылка ]
WEB:: [ Ссылка ]
Recorded at the ACM CHI Conference on Human Factors in Computing Systems in Montréal, Canada April 21-26, 2018
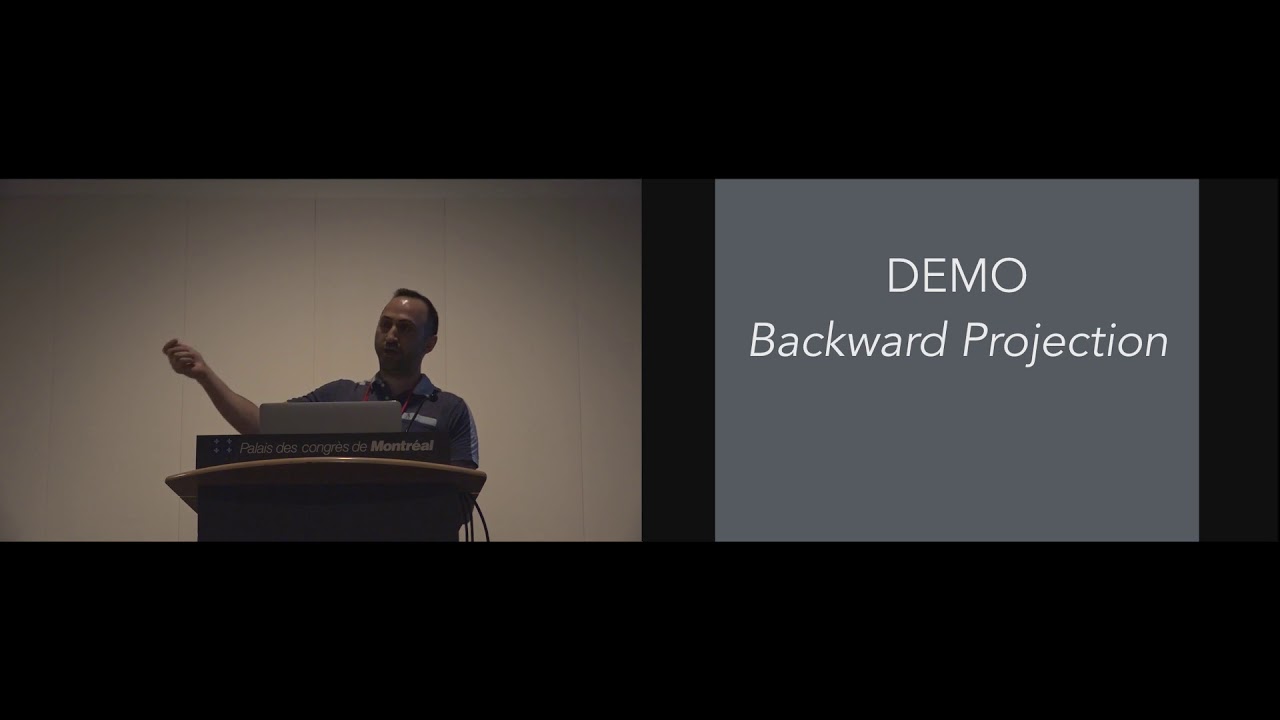