User Trust in Recommendation Systems: A comparison of Content-Based, Collaborative and Demographic Filtering
Mengqi Liao, S. Shyam Sundar, Joseph B. Walther
CHI'22: ACM Conference on Human Factors in Computing Systems
Session: Trust, Recommendation, and Explanable AI (XAI)
Abstract
Three of the most common approaches used in recommender systems are content-based fltering (matching users’ preferences with products’ characteristics), collaborative fltering (matching users with similar preferences), and demographic fltering (catering to users based on demographic characteristics). Do users’ intuitions lead them to trust one of these approaches over others, independent of the actual operations of these diferent systems? Does their faith in one type or another depend on the quality of the recommendation, rather than how the recommendation appears to have been derived? We conducted an empirical study with a prototype of a movie recommender system to fnd out. A 3 (Ostensible Recommender Type: Content vs. Collaborative vs. Demographic Filtering) x 2 (Recommendation Quality: Good vs. Bad) experiment (N=226) investigated how users evaluate systems and attribute responsibility for the recommendations they receive. We found that users trust systems that use collaborative fltering more, regardless of the system’s performance. They think that they themselves are responsible for good recommendations but that the system is responsible for bad recommendations (refecting a self-serving bias). Theoretical insights, design implications and practical solutions for the cold start problem are discussed.
WEB:: [ Ссылка ]
Pre-recorded presentations of CHI 2022
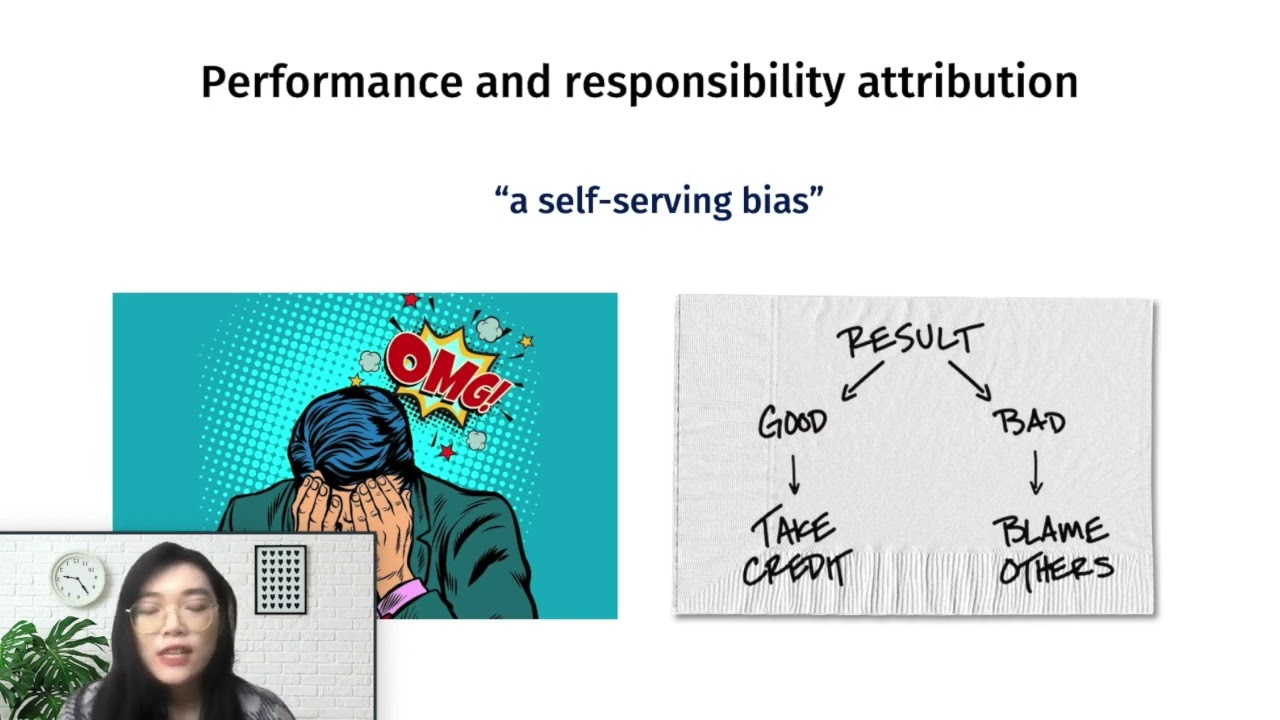