“Absci’s Data and AI Platform for In Silico Antibody Design & Optimization” presented at NVIDIA GTC 2022 by Absci Lead AI Scientist Joshua Meier.
In this presentation Joshua Meier describes two of the company’s drug discovery machine-learning breakthroughs and presents validation of Absci’s in-silico lead optimization models. The validation, requiring wet-lab testing of model predictions, was enabled by Absci’s proprietary and highly engineered synthetic biology platform and assays.
- The first breakthrough is a machine learning model for quantitative prediction of antibody target affinity, allowing computational predictions of binding strength. In one example, Absci demonstrated that this model could accurately predict affinity across four orders of magnitude for previously unseen trastuzumab variants, including accurately predicting variants that had better target affinities than wild-type trastuzumab.
- The second breakthrough is a machine learning model to score ‘naturalness’ of antibody variants; naturalness is a parameter that Absci shows is associated with multiple developability characteristics, and antibodies with better developability have a better likelihood of success as drug candidates through preclinical testing and clinical development.
- Further, Absci showed that generative ML techniques enabled simultaneous in-silico optimization for both affinity and naturalness, underscoring the viability of the computational lead optimization pipeline.
Learn more at [ Ссылка ] and [ Ссылка ]
Join us on social media:
Twitter: [ Ссылка ]
LinkedIn: [ Ссылка ]
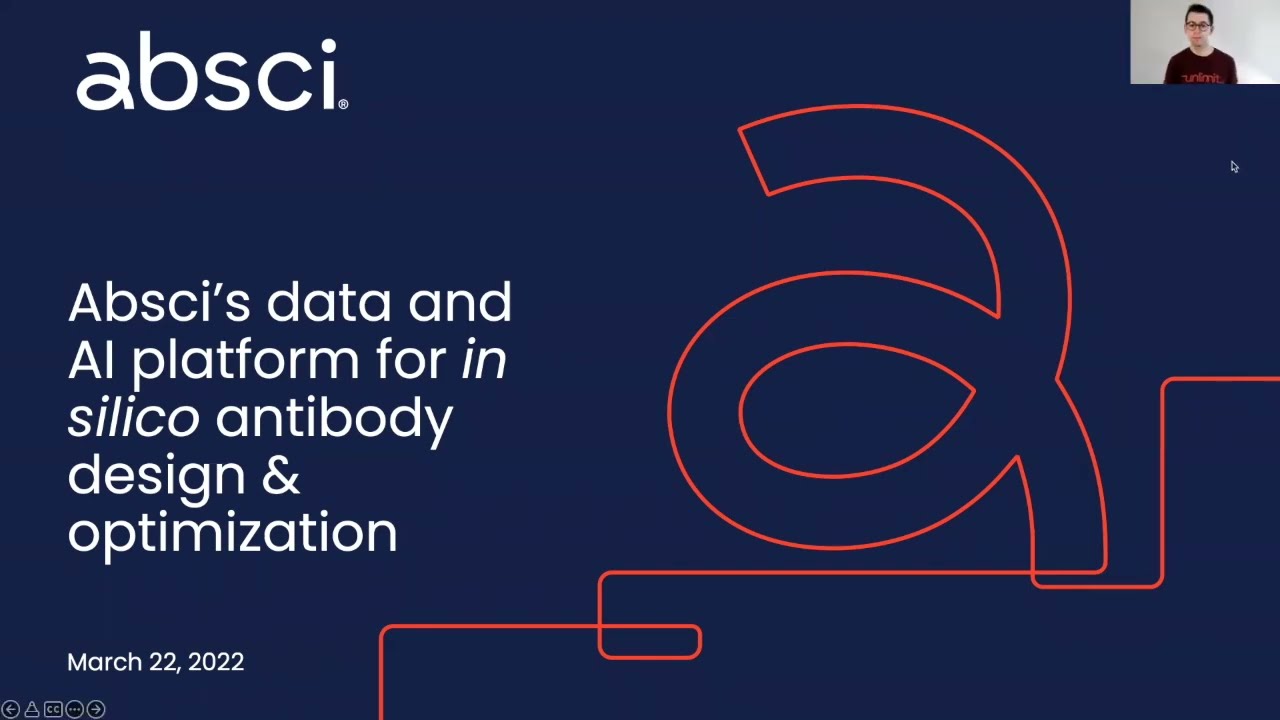