Friday, September 28, 2018
Statistical inference under local information constraints
Abstract:
Independent samples from an unknown probability distribution p on a domain of size k are distributed across n players, with each player holding one sample. Each player can communicate L bits to a central referee, with the goal of resolving a prespecified inference problem. When L greater than or equal to log k bits, the problem reduces to the well-studied centralized case, where all the samples are available in one place. We focus on the communication-starved setting L less than log k, in which as we will see the landscape changes drastically.
We develop a general formulation for inference problems in this distributed setting, and instantiate it for two prototypical inference questions, learning and identity testing. We will consider and discuss the power of shared randomness in distributed inference, and show that for identity testing, schemes without public randomness can be dramatically less efficient than those with.
Finally, we show that our framework is general enough to be extended to other distributed settings, in particular to local differential privacy.
Based on joint works with Clement Canonne, Cody Freitag, and Himanshu Tyagi.
Bio:
Jayadev Acharya is an assistant professor in school of ECE, Cornell University, where he joined after a postdoc at MIT. He holds a Ph.D from UC San Diego. His research interests are in algorithms, information theory, machine learning, and statistics. His received a Jack Wolf Student paper award at ISIT'10, and a Best Paper Honorable Mention at ICML'17.
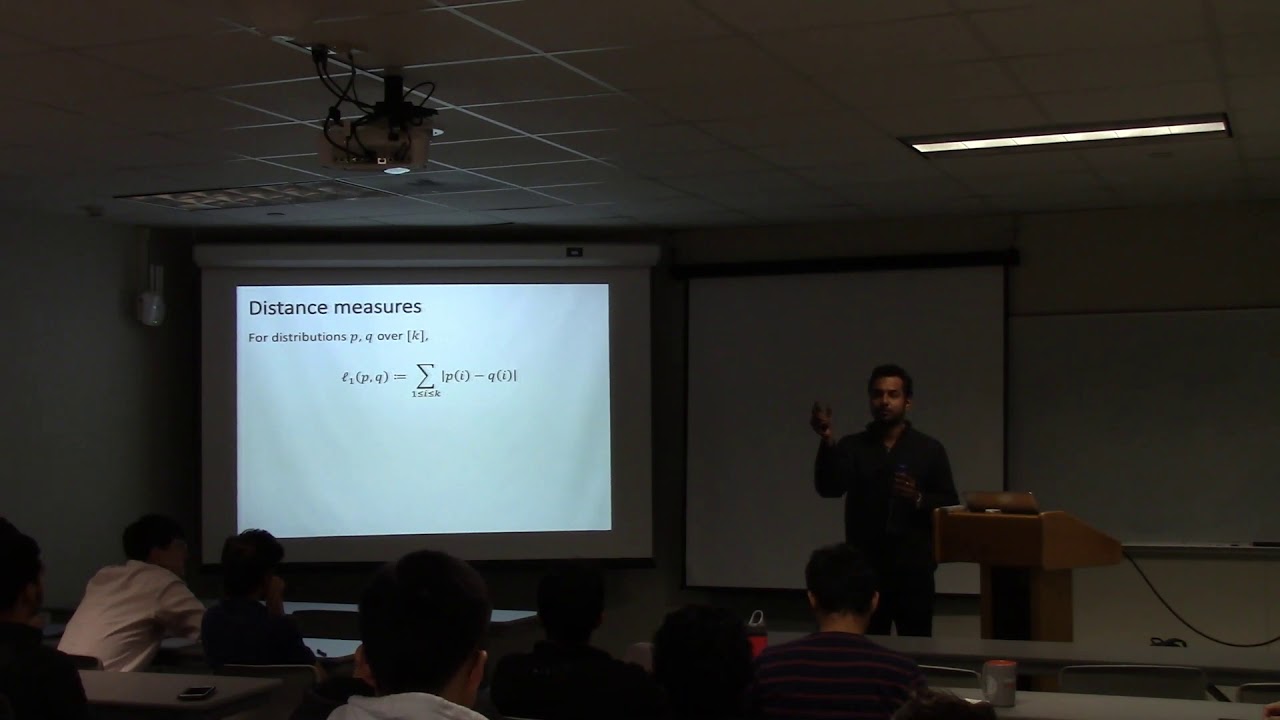