Immense-Scale Machine Learning: The Big, the Small, and the Not Right at All
Norm Jouppi, Google
We start with key aspects of the decade-long evolution of Google’s Tensor Processing Systems. These systems have unique requirements arising from serving billions of daily users across multiple products in production environments. Training Large Language ML Models (LLMs) can require 100,000 accelerators working together in synchrony for months. And LLM inference can require exaFLOP/OP speeds with response times of under a second. This has driven adoption of lower-precision numerics across the industry. Finally, since random errors from various sources are likely to occur at this immense scale during months-long training runs, fault tolerance is also a key feature of immense-scale ML systems.
SUBMIT • ATTEND • VOLUNTEER
[ Ссылка ]
—
SC24 • 17–22 NOV 2024 • ATLANTA, GA
The International Conference for High Performance Computing, Networking, Storage, and Analysis
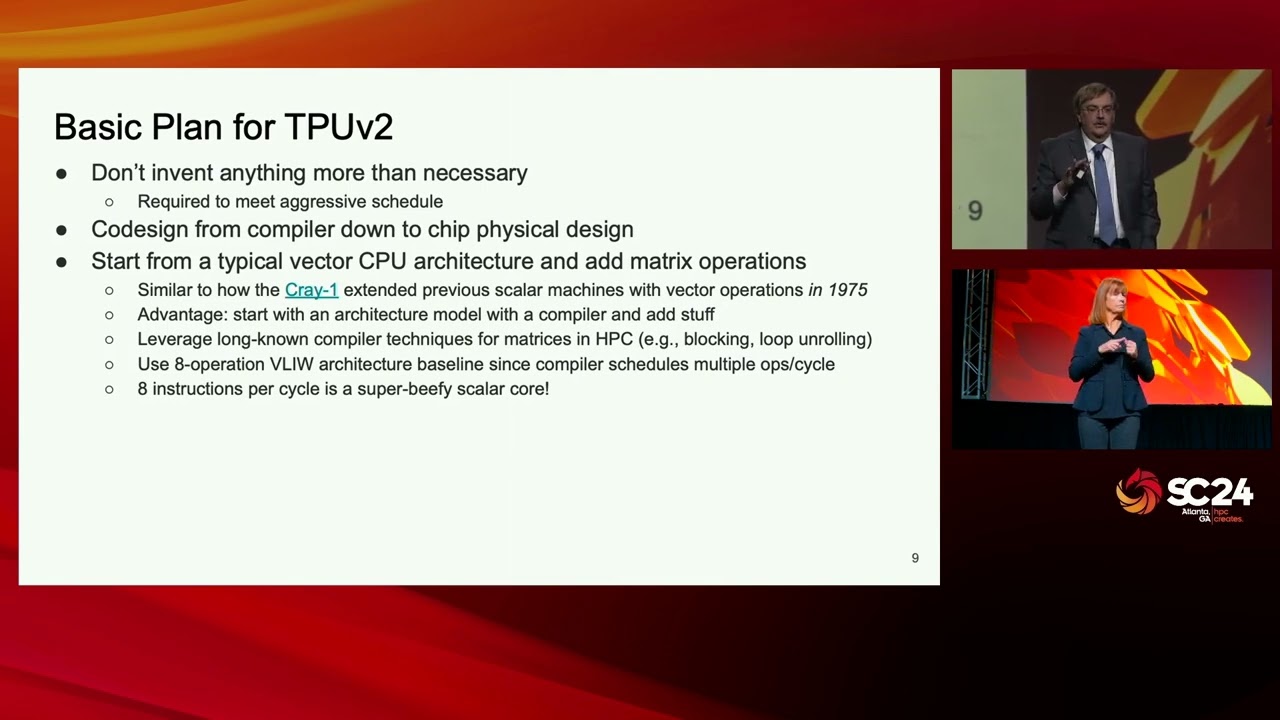