This guest lecture explores the pivotal role of data in training language models, addressing both compute-limited and data-limited regimes. It highlights the impact of data selection, using models' loss measurements to prioritize high-quality training data, and introduces innovative methods like synthetic data augmentation through knowledge graphs to boost efficiency in low-resource domains. By leveraging techniques like rephrasing and injecting diversity, these methods improve performance in niche domains while addressing challenges such as diversity loss and diminishing high-quality internet data. The talk underscores the importance of efficient data utilization for advancing language model capabilities, bridging the gap between pre-training and domain-specific expertise.
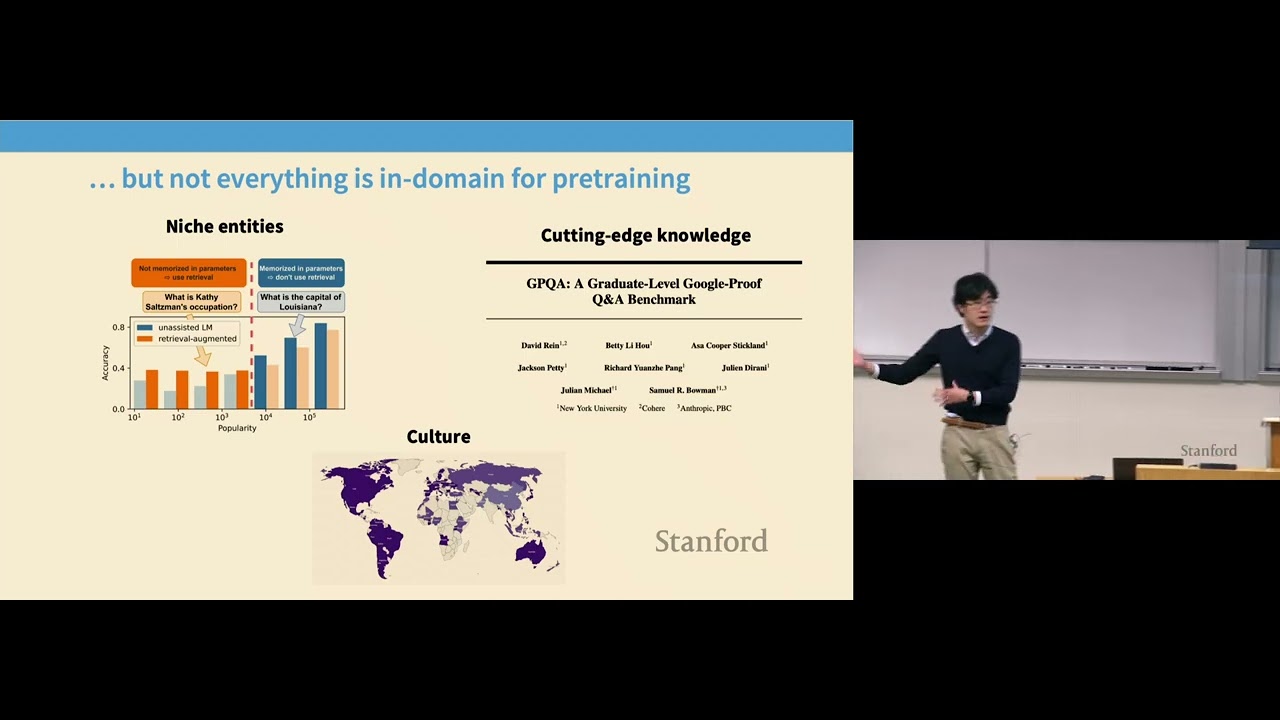