At the 2018 American Society of Hematology (ASH) Annual Meeting in San Diego, Aziz Nazha, MD of Cleveland Clinic discussed the results of a personalized prediction model for Myelodysplastic Syndromes (MDS). Dr. Nazha and colleagues reported that the model outperformed current prognostic scoring systems in predicting an MDS patient's risk of mortality and transformation to acute myeloid leukemia (AML), a more aggressive type of bone marrow cancer.
Patients with MDS have survival outcomes that can range from months to decades. Although several prognostic scoring systems have been developed to risk stratify MDS patients, survival varies even within distinct categories, which may lead to over- or under-treatment. Researchers hypothesized that discrepancies may be due to analytic approaches or lack of incorporation of molecular data. The machine learning model outperformed International Prognostic Scoring System (IPSS) and Revised IPSS (IPSS-R) in predicting survival outcomes and risk for acute myeloid leukemia (AML) transformation among a training cohort of 1471 patients.
In addition, the researchers conducted several feature extraction analyses to identify the most important variables that impacted patients’ outcomes, as well as the least number of variables that produced the best prediction. From most important to least, variables included cytogenetic risk categories by IPSS-R, platelets, mutation number, hemoglobin, bone marrow blast percentage, 2008 World Health Organization diagnosis, white blood cell count, age, absolute neutrophil count (ANC), absolute lymphocyte count (ALC), TP53, RUNX1, STAG2, ASXL1, absolute monocyte counts (AMC), SF3B1, SRSF2, RAD21, secondary versus de novo MDS, NRAS, NPM1, TET2, and EZH2.
Conclusion
Dr. Nazha and colleagues built a personalized prediction model based on clinical and genomic data that outperformed IPSS and IPSS-R in predicting OS and AML transformation. The new model gives survival probabilities at different time points that are unique for a given patient Incorporating clinical and mutational data outperformed a mutations only model even when cytogenetics and age were added.
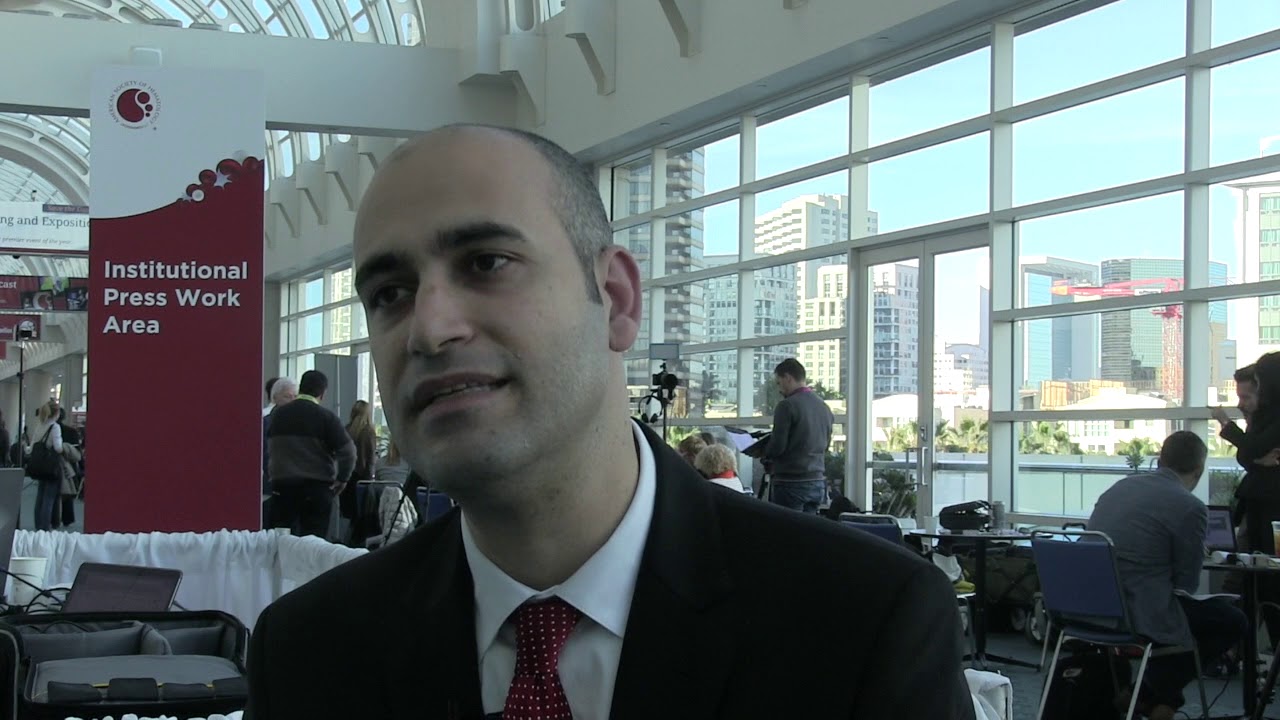