Presenter: Sreelekha Giggilam, MS Computational Data Science student at the University at Buffalo
This video was submitted as part of the STEM for Everyone initiative hosted by the UB Women in STEM Cooperative (WISC) and UB Women in Science and Engineering (WiSE). Learn more at www.buffalo.edu/wise/stemforeveryone.
Abstract: The identification of extreme COVID-19 trends as well as the policies that impact COVID-19 infections across countries is crucial and often complex. The large scale and dimensionality of the COVID time series data (cases, deaths, recoveries, multiple policies, etc.) along with its temporal dependency makes the problem challenging. Thus, we propose an online anomaly detection method to identify anomalies in a collection of multivariate time series using large deviations principle. We demonstrate its applicability in identifying anomalous trends in COVID-19 infections and the significance of different interventions and policies in each country.
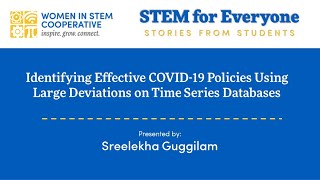