The sources discuss emerging technologies in the context of data strategy, focusing on their impact on various industries and providing implementation guidelines.Emerging technologies are reshaping data strategy across industries, requiring organizations to adapt their infrastructure, skills, and processes to leverage these advancements effectively. Some of the key technologies and trends discussed include:
* Edge Computing: Edge computing enables distributed processing and local intelligence, which is particularly crucial for IoT integration. This allows for faster data processing, reduced latency, and improved privacy by keeping sensitive data closer to its source.
* Quantum Computing: Quantum computing has the potential to revolutionize complex optimization problems, enhance security measures, and provide unprecedented simulation capabilities. Although still in its early stages, quantum computing could solve problems currently intractable for classical computers.
* Sustainable Computing: Driven by environmental concerns, there is a growing focus on green AI and energy-efficient computing. This trend includes developments in energy-efficient hardware and algorithms, as well as carbon management strategies for data centers.
* Federated Learning: This approach allows machine learning models to be trained across multiple decentralized devices or servers holding local data samples, without exchanging them. This addresses privacy concerns and enables collaboration on sensitive data.
The sources emphasize the strategic implications of these trends, arguing that organizations need to address both technology integration and organizational adaptation to effectively navigate the evolving data landscape.
* Technology integration involves designing flexible, scalable systems that can incorporate emerging technologies, ensuring seamless communication between diverse data sources and analytics tools, establishing robust frameworks for data quality, privacy, and compliance, and implementing advanced security measures to protect against evolving cyber threats.
* Organizational adaptation requires investing in training and recruitment to build expertise in emerging technologies, reengineering business processes to leverage new data capabilities, fostering a data-driven culture that embraces innovation and continuous learning, and implementing strategies to guide the organization through technological transitions.
Key research findings from sources like the Stanford Digital Economy Lab and McKinsey Global Institute highlight the impact of emerging technologies on market structures and the need for organizations to adapt their strategies and capabilities:
* Competition is becoming increasingly platform-based with data-driven ecosystems becoming central to market dynamics.
* Innovation is accelerating, driven by advancements in artificial intelligence and machine learning technologies.
* Value creation is expanding through network effects, emphasizing the importance of data network and ecosystem strategies.
* Governance structures are evolving to become more risk-aware, addressing the complex challenges posed by data privacy, security, and ethical considerations.
The sources provide implementation guidelines and frameworks for organizations to successfully adopt emerging technologies:
* Risk Management: McKinsey Global Institute research emphasizes comprehensive risk management when implementing data strategies, highlighting technical, operational, strategic, and compliance risks. They provide a risk management matrix to identify, mitigate, and monitor these risks.
* Capability Requirements: MIT CISR research stresses the development of robust technical, organizational, and strategic capabilities. These include infrastructure modernization, integration architecture, security frameworks, process optimization, governance structures, skill development, change management, innovation management, value creation, market positioning, and risk management.
* Implementation Methodology: Stanford Digital Economy Lab research recommends a phase-driven approach for implementation, including assessment, design, implementation, optimization, and evolution. This cyclical approach emphasizes continuous improvement and adaptation in data strategy implementation.
* Innovation Management: Stanford research suggests creating dedicated environments for testing and validating new technologies, fostering rapid prototyping, and establishing mechanisms to rapidly scale successful innovations.
* Future Value Creation Models: MIT CISR research proposes a framework for understanding how emerging technologies can drive value creation in organizations, differentiating between traditional, innovative, disruptive, and evolutionary models.
* Competitive Positioning: Carnegie Mellon research identifies key positioning strategies for organizations to gain a competitive advantage in the evolving technological landscape, including innovation leadership,...
...
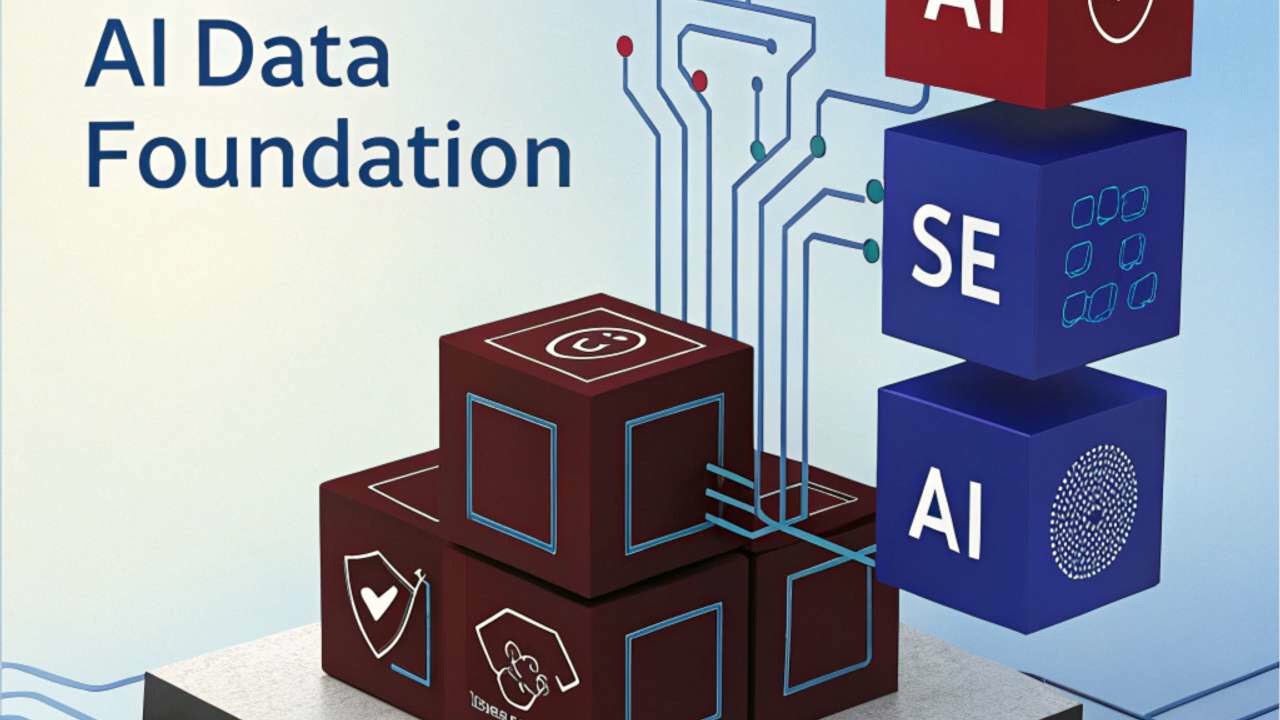