How can we train a robot that can generalize to perform thousands of tasks in thousands of environments? This question underscores the holy grail of robot learning, more generally machine learning, research. Current AI systems are incredibly specific in that they only perform the tasks they are trained for and are miserable at generalization. One fundamental reason is that these systems are trained once and kept fixed at inference, thus making it difficult to generalize to scenarios far from the ones seen in training data. In contrast, generalization in humans stems from our ability to explore and adapt continually throughout our lifetime. In this talk, I will present our early efforts that draw ideas from human learning to build a continually adaptive robotic framework. I will focus on three key questions: (1) how to continually generate supervision for oneself (curiosity); (2) how to bootstrap the learning by observing other humans (social learning); and (3) how to adapt already learned skills in real-time (adaptation). I will demonstrate the potential of this framework for scaling up robot learning via case studies of robots discovering new tasks in complex kitchen environments, controlling dextrous robotic hands from monocular vision, robotics walking on unseen terrains, and performing lots of diverse manipulation tasks in the wild.
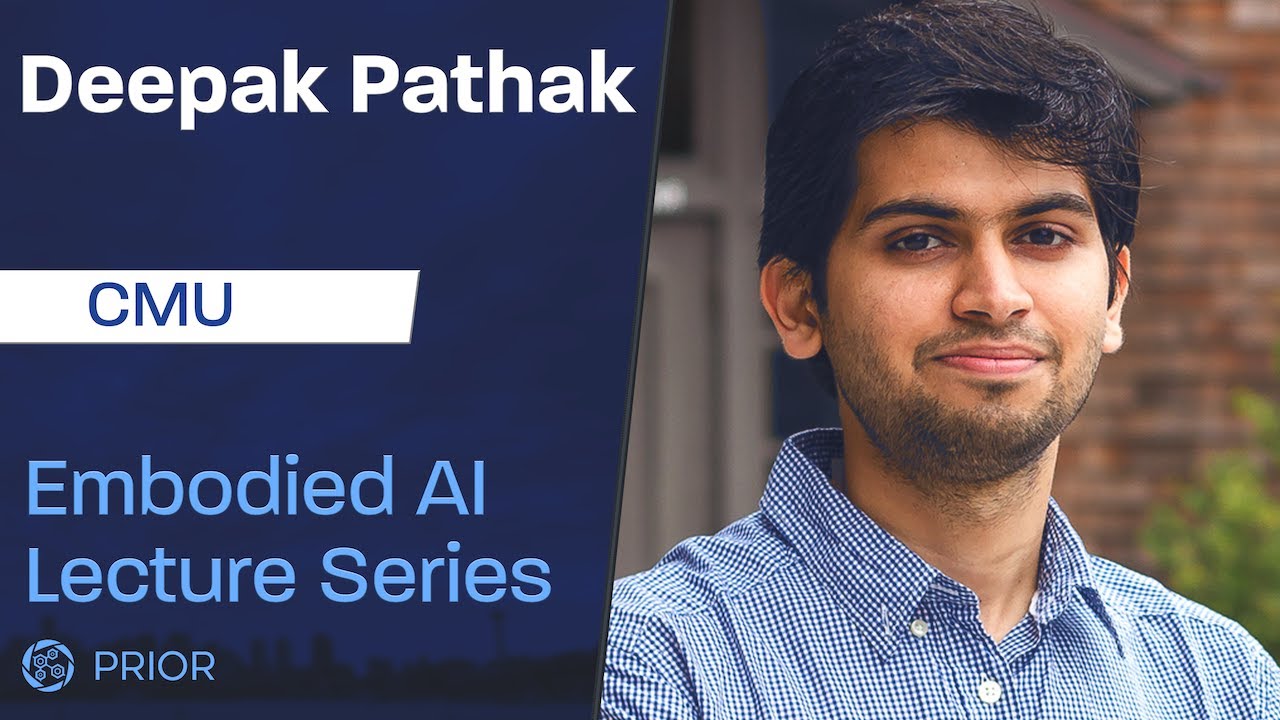