6th Machine Learning and AI in Bio(Chemical) Engineering Conference (MABC)
06/July/2023
For more information: [ Ссылка ]
Abstract:
One of the main aims of enzymatic biocatalysis is to replace conventional chemical synthesis byoffering more sustainable catalytic alternatives (solvent, temperature, etc.) for key stages in the processes. For this, it is essential to find the most efficient enzyme for the given set of conditions,and since the molecules synthesized rarely have optimized natural biosynthesis pathways, it is crucial to be able to seek new enzymes with improved activity and selectivity. Historically, there have been two opposing approaches: enzyme engineering or biodiversity exploration. Although they have proved effective to date, both are a posteriori method, since it is still impossible to predict an enzyme's activity from its peptide sequence alone. That said, the rapid emergence of machine learning (ML) in this field, such as the Alphafold "revolution" [1], is changing this paradigm, and several studies are beginning to move towards this goal [2–7]. The main limitationthat seems to remain is the availability of robust and curated experimental datasets describing enzyme activity for a given family, with most studies relying heavily on the often highly heterogeneous data available in international databases. That's why in the present study we wereinterested in exploiting our recent dataset around the transaminase family [8,9]. This dataset, comprising more than 25,000 activity assays performed under the same experimental conditions,on more than twenty different substrates, was generated a few years ago using a new highthroughput screening strategy to identify new transaminases suitable for synthesis. To achieve our objective, we began by attempting to correlate enzyme sequences with their activity for different substrates using neural networks. Some of the tested architectures proved effective in solving this problem once transformed into a classification problem, by grouping activities into 4 major classes. However, the high proportion of weak enzyme activities in the dataset seemed tolimit the prediction accuracy for a regression-type approach. With this in mind, we decided to introduce more information at enzyme level, to establish finer correlations between their active site,substrates and activities. For this, and inspired by some recent studies using docking [2] andGNN [5,10], we started designing a new workflow which will be detailed in this talk and that is based on several ML-based available tools (Colabfold, P2Rank, Gnina, BagPype). It aims at 1) predicting the structure of our enzymes, 2) at docking the different substrates and co-factors inside the latter, and 3) at transforming the resulting 3D file into a network visualization that could be used as additional input to our neural networks.
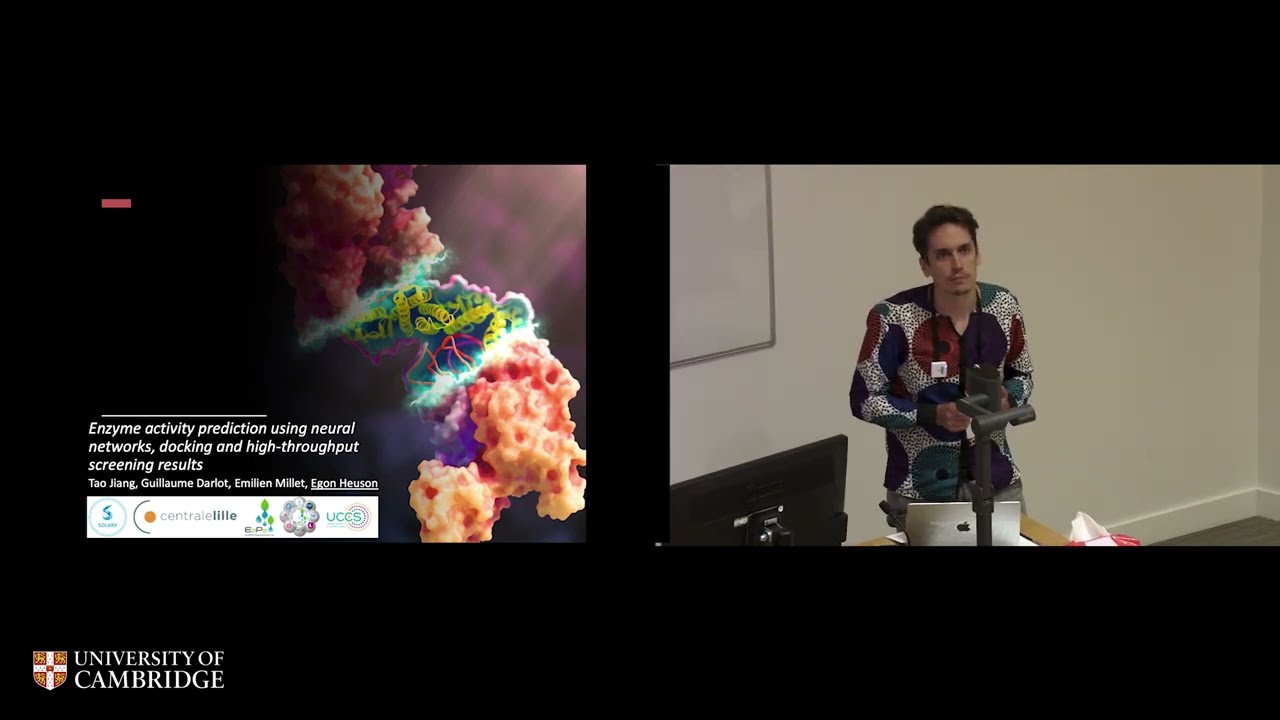