Spintronic Devices for Artificial Neural Networks
This online seminar was given on August 14, 2020, by Dr. Saima Siddiqui of the University of Illinois at Urbana-Champaign. Please refer to [ Ссылка ] for more information about the Online Spintronics Seminar Series.
Abstract:
Spintronics promises intriguing device paradigms where electron spin is used as the information token instead of its charge counterpart. In the future cognitive era, nonvolatile magnetic memories hold the key to solve the bottleneck in the computational performance due to data shuttling between the processing and the memory units. The application of spintronic devices for these purposes requires versatile, scalable device design that is adaptable to emerging material physics. We design, model and experimentally demonstrate spin orbit torque induced magnetic domain wall devices as the building blocks (i.e. linear synaptic weight generator and the nonlinear activation function generator) for in-memory computing, in particular for artificial neural networks. Spin orbit torque driven magnetic tunnel junctions show great promise as energy efficient emerging nonvolatile logic and memory devices. In addition to its energy efficiency, we take advantage of the spin orbit torque induced domain wall motion in magnetic nanowires to demonstrate the linear change in resistance of the synaptic devices. Modifying the spin-orbit torque from a heavy metal or utilizing the size dependent magnetoresistance of tunnel junctions, we also demonstrate a nonlinear activation function for thresholding signals (analog or digitized) between layers for deep learning. A complete neuromorphic hardware accelerator using embedded nonvolatile magnetic domain wall devices can revolutionize computer architectures by embedding memory into logic circuits in a fine grained fashion.
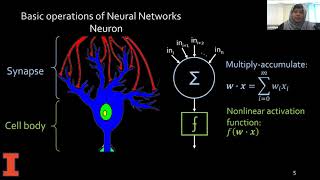