As acquiring pixel-wise annotations of real-world images for semantic segmentation is costly, a model can instead be trained with more accessible synthetic data and adapted to real images without requiring their annotations. This process is studied in unsupervised domain adaptation. Most research in these areas focused on the design of adaptation strategies to overcome the problem of the domain gap.
Lukas Hoyer’s talk focuses on the influence of secondary factors on the overall success of domain adaptation, such as network architecture, general training strategies, image resolution, crop size, and image masking.
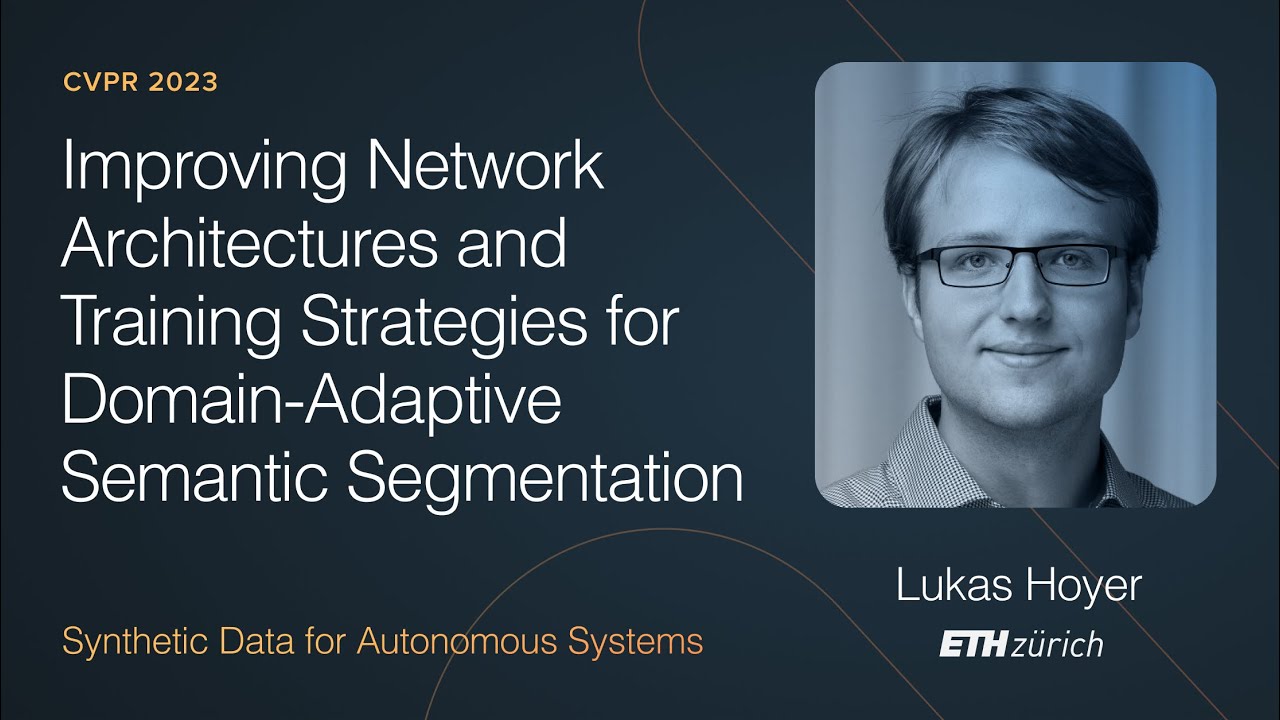