Efficient navigation through uneven terrain remains a challenging endeavor for autonomous robots. We propose a new geometric-based uneven terrain mapless navigation framework combining a Sparse Gaussian Process (SGP) local map with a Rapidly-Exploring Random Tree* (RRT*) planner. Our approach begins with the generation of a high-resolution SGP local map, providing an interpolated representation of the robot's immediate environment. This map captures crucial environmental variations, including height, uncertainties, and slope characteristics. Subsequently, we construct a traversability map based on the SGP representation to guide our planning process. The RRT* planner efficiently generates real-time navigation paths, avoiding untraversable terrain in pursuit of the goal. This combination of SGP-based terrain interpretation and RRT* planning enables ground robots to safely navigate environments with varying elevations and steep obstacles. We evaluate the performance of our proposed approach through robust simulation testing, highlighting its effectiveness in achieving safe and efficient navigation compared to existing methods. We have released our source code at [ Ссылка ].
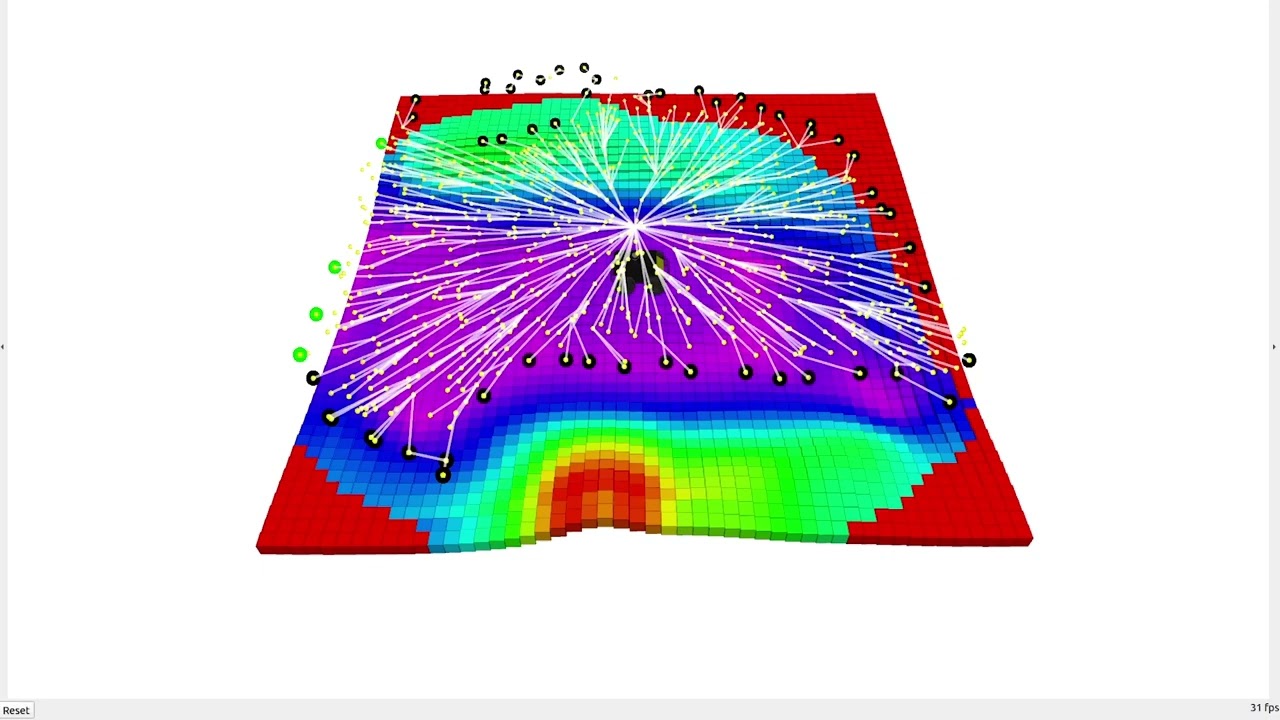