Title: Reinforcement Learning-based Grasping via One-Shot Affordance Localization and Zero-Shot Contrastive Language–Image Learning
Authors: Xiang Long, Luke Beddow, Denis Hadjivelichkov, Andromachi Maria Delfaki, Dimitrios Kanoulas
Venue: 16th IEEE/SICE International Symposium on System Integration (SII)
Project page: [ Ссылка ]
PDF: [ Ссылка ]
Abstract: Legged robots, particularly quadrupeds, offer promising navigation capabilities, especially in scenarios requiring traversal over diverse terrains and obstacle avoidance. This paper addresses the challenge of enabling legged robots to navigate complex environments effectively through the integration of data-driven path-planning methods. We propose an approach that utilizes differentiable planners, allowing the learning of end-to-end global plans via a neural network for commanding quadruped robots. The approach leverages 2D maps and obstacle specifications as inputs to generate a global path. To enhance the functionality of the developed neural network-based path planner, we use Vision Transformers (ViT) for map pre-processing, to enable the effective handling of larger maps. Experimental evaluations on two real robotic quadrupeds (Boston Dynamics Spot and Unitree Go1) demonstrate the effectiveness and versatility of the proposed approach in generating reliable path plans.
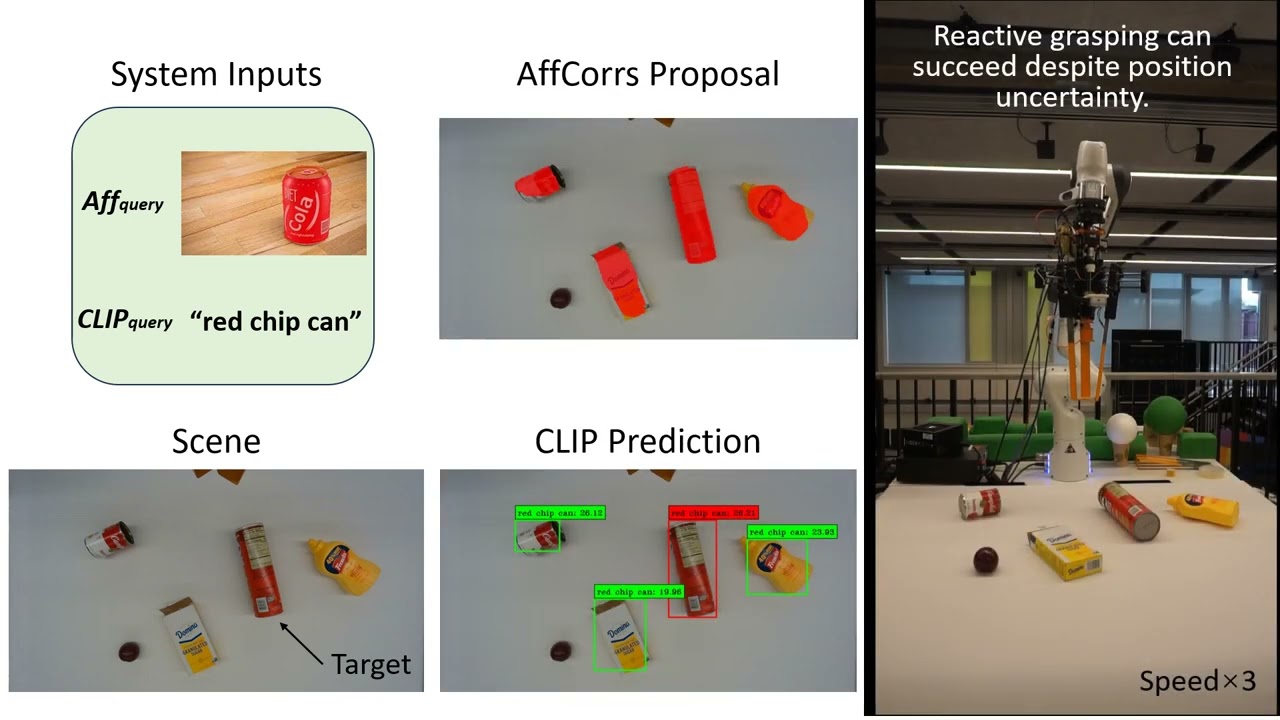