In this lecture, I'll turn my philosophical gaze on the actual process — the activity — of doing science. Previously, I've tried to convince you that new knowledge (a) must be built on what is already known, (b) depends on the individual perspectives of researchers, and (c) will always continue to change and expand our view of the world. We've also already agreed that knowledge is not limited to language, but includes tacit elements such as practical know-how, personal judgments, and passionate commitments (what we care about), which are all not entirely under our rational or even volitional control.
Here, I ask what kind of epistemology we can develop to understand the quality of our scientific activities given this "irrational underbelly" or scientific research. Knowledge can only be understood in connection with the process that generates it. There is no general (or a priori) epistemology. Our aim must be to understand which processes can reliably generate high-quality insights that allow us to solve our particular problems and to satisfy our particular epistemic goals.
I use the work of philosopher Philip Kitcher to show how we can appraise the quality of our cognitive performance as researchers. The aim of his naturalistic epistemology is to learn from successful scientific practices (and errors) in the past to achieve a structured account of reality. As Aristotle put it: science attempts to describe the order of things. In fact, the history of science can be seen as the history of such attempts. It indicates that we are getting better and better at it. While we cannot come up with any general recipe to get an objective understanding of reality, we can at least understand which strategies are best to achieve our epistemic goals in a given context. This kind of epistemology may be less impressive than classical or positivist attempts at reaching universal knowledge, but at least it works in the world with limited human beings as they actually are.
To illustrate what I mean by epistemic goals and different epistemic strategies, we look at Thomas Kuhn's essential tension between productive tradition and risky innovation in science. Which strategy works best under which circumstances? This tension can be described in computer-science terms through explore-exploit strategies to problem solution. Any attempt to frame scientific investigation as a rational process will be biased towards exploitation, which is dominating our current academic system. I would argue that in our current situation we urgently need to (re)learn how to explore again!
In the next (and final) lecture of this series, we will be looking at the social aspects of doing science.
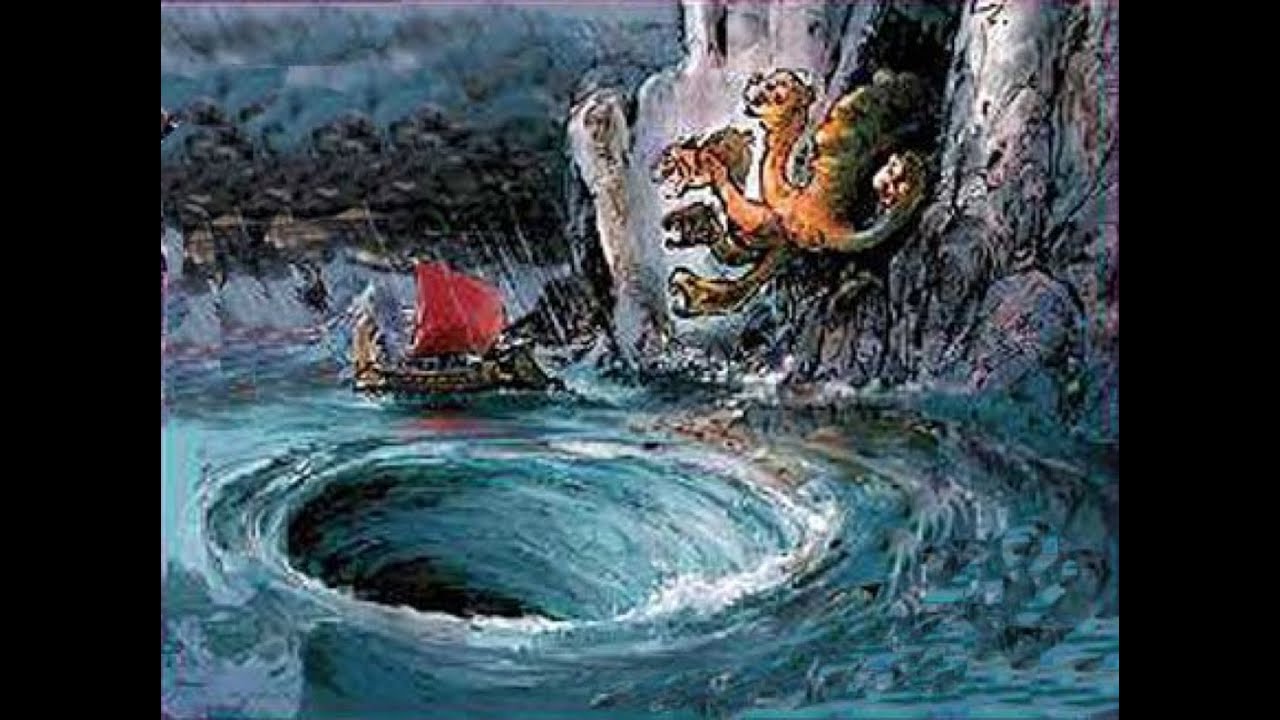