Speaker: Thomas G. Dietterich (Oregon State University)
Abstract: This talk will review the basic methods for anomaly detection in both "feature vector" data and in image data. It will present evidence that the factors underlying the performance in these two areas differ. Anomalies in feature vector data are typically outliers, whereas anomalies in deep learning are inliers. This suggests that deep anomaly detection is inherently more brittle than anomaly detection on feature vector data.
Speaker's Bio:
Dr. Dietterich (AB Oberlin College 1977; MS University of Illinois 1979; PhD Stanford University 1984) is Distinguished Professor Emeritus in the School of Electrical Engineering and Computer Science at Oregon State University. Dietterich is one of the pioneers of the field of Machine Learning and has authored more than 225 refereed publications and two books. His current research topics include robust artificial intelligence, robust human-AI systems, and applications in sustainability.
Dietterich has devoted many years of service to the research community. He is a former President of the Association for the Advancement of Artificial Intelligence, and the founding president of
the International Machine Learning Society. Other major roles include Executive Editor of the journal Machine Learning, co-founder of the Journal for Machine Learning Research, and program chair of AAAI 1990 and NIPS 2000. He currently serves as one of the moderators for the cs.LG category on arXiv.
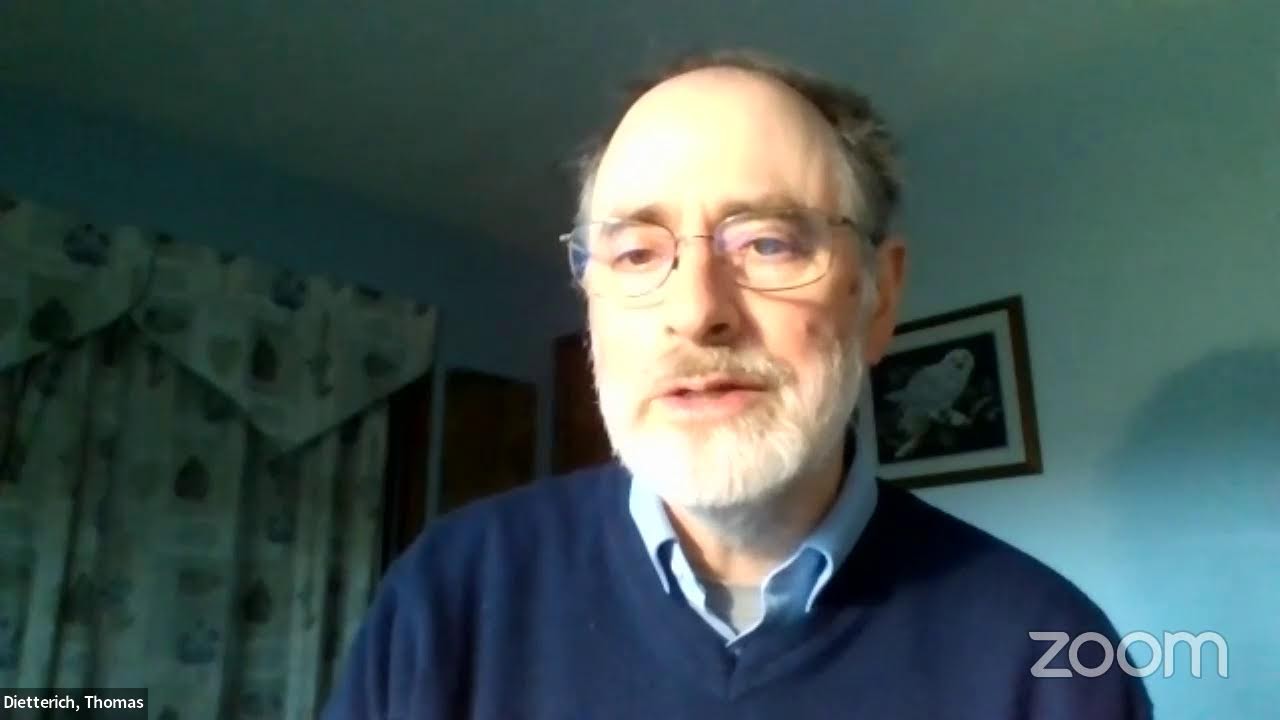