This is a talk delivered at the (usually not recorded) weekly journal club "Deep Learning: Classics and Trends" ([ Ссылка ] ).
Speaker: Muhammad Khalifa
Title: Discriminator-Guided Chain-of-Thought Reasoning
Abstract: During this talk, we'll explore the challenges Large Language Models (LLMs) face with chain-of-thought (multi-step) reasoning, often leading them to invalid solutions when using standard decoding techniques. As LLMs can assign a high probability to incorrect reasoning steps and vice versa, decoding techniques that optimize for sequence probability can easily produce incorrect reasoning. I will begin the talk by discussing the issues with standard decoding techniques in reasoning and the limitations of post hoc approaches such as self-consistency and verifiers. Then I will introduce GRACE—a guided decoding method that leverages a specially trained discriminator to guide the LLM decoding toward correct reasoning steps. We'll show that GRACE can boost the reasoning of LLMs on mathematical and symbolic tasks, producing not just correct final answers but also reliable reasoning chains, while outperforming standard decoding and post hoc techniques. The talk will conclude with a discussion on the limitations and future directions for inference-time methods to advance LLM reasoning.
Speaker bio: Muhammad Khalifa is a third-year Ph.D. candidate at the University of Michigan in Ann Arbor, advised by Honglak Lee and Lu Wang. Muhammad's research interests involve reasoning with LLMs, RL for language, and LLM attribution. Muhammad has done research internships at NAVER Labs Europe, Amazon AWS, and the Allen Institute for AI.
Paper link: [ Ссылка ]
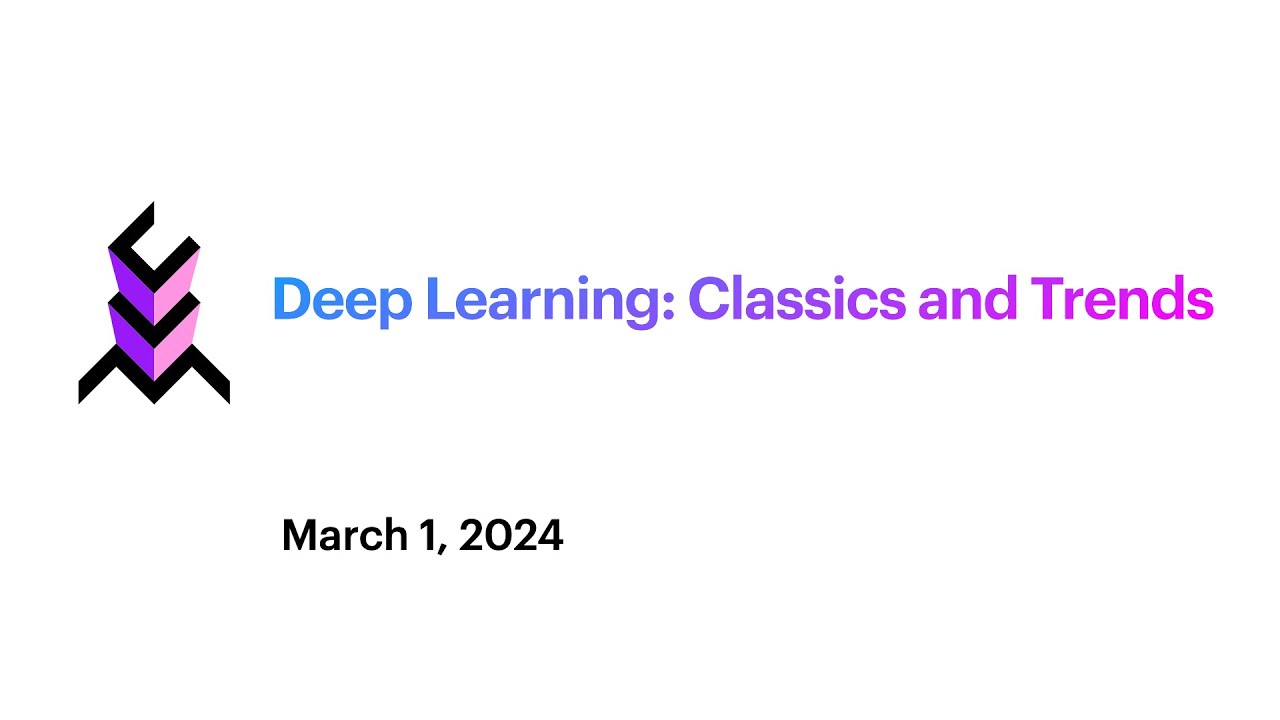