Investigating the Effect of Sensor Data Visualization Variances in Virtual Reality
Thanh Vu, Dinh Tung Le, Dac Dang Khoa Nguyen, Sheila Sutjipto, and Gavin Paul
VRST 2021
Session: Paper 2: Visualization
Abstract
This paper investigates the effect that variances in real-time sensor data, such as resolution, compression and frame-rate, have on humans performing straightforward assembly tasks in a Virtual Reality-based (VR-based) training system. A VR-based training system has been developed that transfers RGB and depth image and constructs colored point clouds data to represent objects in front of a participant. Various parameters that affect sensor data acquisition and visualization of remotely operated robots in the real-world are varied, and the associated task performance is observed. Experimental results conducted by 12 participants performing a cumulative total of 95 VR-guided puzzle assembly tasks showed that a combination of low resolution and uncolored points has the most significant effect on participants' performance, where an increase in both stress and time was observed. Participants mentioned that they needed to rely upon tactile feedback when the pure perceptual feedback was unhelpful. The most insignificant parameter was found to be the resolution of the image and point cloud, which, when varied within the experimental bounds, only resulted in a 5% average change in completion time. Participants also indicated in surveys that they felt their performance had improved, and frustration was reduced when given the color data compared to when they received a point cloud without the true color applied.
DOI:: [ Ссылка ]
WEB:: [ Ссылка ]
Videos for VRST 2021
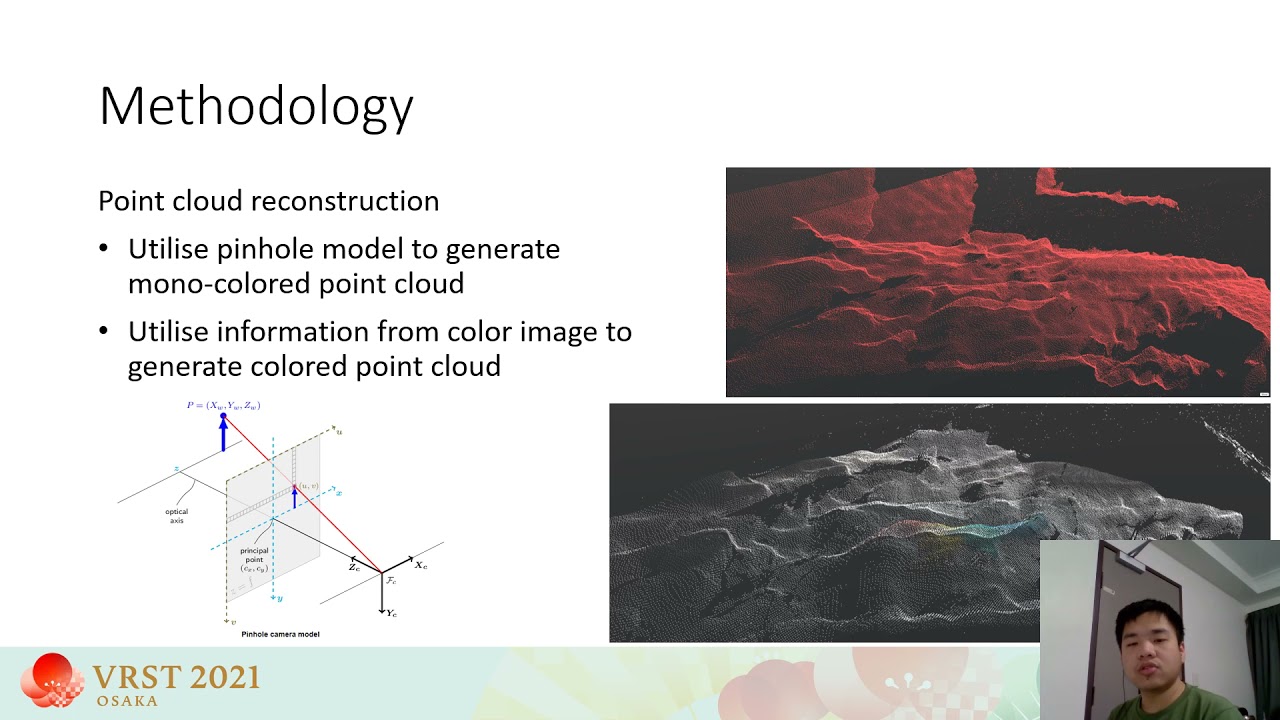