**Emergent Real-World Robotic Skills via Unsupervised Off-Policy Reinforcement Learning**
Archit Sharma (Google)*; Michael Ahn (Google); Sergey Levine (Google); Vikash Kumar (Google); Karol Hausman (Google Brain); Shixiang Gu (Google Brain)
Publication: [ Ссылка ]
webpage: [ Ссылка ]
supplementary video: [ Ссылка ]
**Abstract**
Reinforcement learning provides a general framework for learning robotic skills while minimizing engineering effort. However, most reinforcement learning algorithms assume that a well-designed reward function is provided, and learn a single behavior for that single reward function. Such reward functions can be difficult to design in practice. Can we instead develop efficient reinforcement learning methods that acquire diverse skills without any reward function, and then re-purpose these skills for downstream tasks? In this paper, we demonstrate that a recently proposed unsupervised skill discovery algorithm can be extended into an efficient off-policy method, making it suitable for performing unsupervised reinforcement learning in the real world. Firstly, we show that our proposed algorithm provides substantial improvement in learning efficiency, making reward-free real-world training feasible. Secondly, we move beyond the simulation environments and evaluate the algorithm on real physical hardware. On quadrupeds, we observe that locomotion skills with diverse gaits and different orientations emerge without any rewards or demonstrations. We also demonstrate that the learnt skills can be composed using model predictive control for goal-oriented navigation, without any additional training.
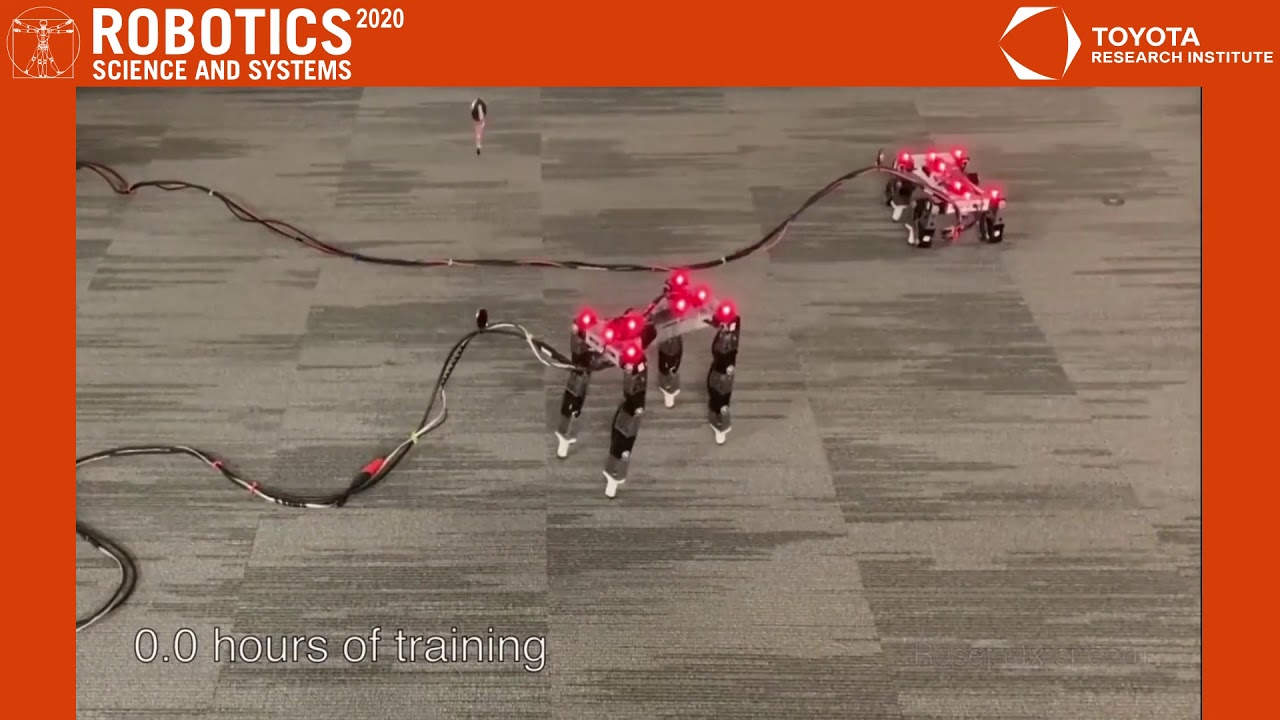